Top 30 Data Quality Analyst Interview Questions and Answers [Updated 2025]
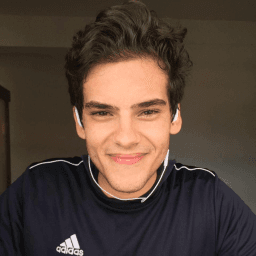
Andre Mendes
•
March 30, 2025
Navigating a Data Quality Analyst interview can be challenging, but preparation is key. In this post, we dive into the most common interview questions you'll encounter for this role. With detailed example answers and insightful tips, we aim to equip you with the tools needed to respond confidently and effectively. Whether you're a seasoned pro or a newcomer, this guide is your roadmap to success.
Download Data Quality Analyst Interview Questions in PDF
To make your preparation even more convenient, we've compiled all these top Data Quality Analystinterview questions and answers into a handy PDF.
Click the button below to download the PDF and have easy access to these essential questions anytime, anywhere:
List of Data Quality Analyst Interview Questions
Behavioral Interview Questions
Can you describe a time when you identified a significant data quality issue? How did you address it?
How to Answer
- 1
Start with a specific incident highlighting the issue.
- 2
Explain the impact of the data quality issue on the business or project.
- 3
Detail the steps you took to investigate and resolve the issue.
- 4
Mention any tools or methods you used during the process.
- 5
Conclude with the outcome and any lessons learned.
Example Answers
In my previous role, I noticed discrepancies in customer data during a routine audit. This affected targeted marketing efforts and resulted in missed campaigns. I gathered the data, used SQL for analysis, and found duplicate entries. After cleaning the data and implementing validation rules, we improved our targeting and saw a 20% increase in campaign effectiveness.
Tell me about a successful collaboration with stakeholders to improve data quality. What was your role?
How to Answer
- 1
Identify the project or initiative for improving data quality
- 2
Describe your specific role and responsibilities in the collaboration
- 3
Highlight the stakeholders involved and their contributions
- 4
Explain the outcome or impact of the collaboration on data quality
- 5
Use metrics or specific results to illustrate success
Example Answers
In a recent project, I led a cross-functional team to clean customer data inconsistencies. I worked with the sales and marketing teams to identify pain points and prioritize quality issues. Together, we implemented a new validation process, resulting in a 30% reduction in errors.
Don't Just Read Data Quality Analyst Questions - Practice Answering Them!
Reading helps, but actual practice is what gets you hired. Our AI feedback system helps you improve your Data Quality Analyst interview answers in real-time.
Personalized feedback
Unlimited practice
Used by hundreds of successful candidates
Describe a situation where you had to adapt to a sudden change in data quality standards. How did you handle it?
How to Answer
- 1
Identify a specific instance where data quality standards changed.
- 2
Explain the context and importance of the change.
- 3
Describe the steps you took to respond to the new standards.
- 4
Highlight the results of your actions and any improvements made.
- 5
Emphasize skills used, like problem-solving and teamwork.
Example Answers
In my previous role, we faced a sudden increase in compliance regulations impacting our data standards. I quickly organized a team meeting to discuss the changes and realigned our data validation protocols. We updated our quality checks and trained our staff, leading to a 30% decrease in errors after implementation.
Have you ever led a team project focused on data quality improvement? What challenges did you face?
How to Answer
- 1
Start with a brief overview of the project and your role as a leader
- 2
Highlight specific data quality issues you aimed to address
- 3
Discuss the team dynamics and how you motivated team members
- 4
Mention key challenges faced like resistance or data discrepancies
- 5
Conclude with the outcomes and improvements achieved in data quality
Example Answers
I led a project to improve customer database accuracy. We identified duplicate entries as a major issue. My role was to align the team on the goal and facilitate weekly discussions. A challenge was initial pushback from some team members over changing existing processes. Ultimately, we reduced duplicates by 40%, significantly enhancing our data quality.
Can you provide an example of a proactive measure you took to enhance data quality in a previous role?
How to Answer
- 1
Think of a specific situation where you identified a data quality issue.
- 2
Describe the proactive steps you took to prevent the problem.
- 3
Mention the tools or methods you used in your approach.
- 4
Include the positive outcome or impact your actions had.
- 5
Keep the response focused and to the point.
Example Answers
In my previous role, I noticed discrepancies in customer data across our databases. I implemented a regular data validation process using automated scripts, which reduced errors by 30% within three months.
Give an example of how you effectively communicated complex data quality issues to non-technical stakeholders.
How to Answer
- 1
Identify a specific data quality issue you handled.
- 2
Use simple language and avoid technical jargon.
- 3
Explain the impact of the issue on the business or stakeholders.
- 4
Suggest actionable steps taken to resolve the issue.
- 5
Highlight any positive outcomes resulting from your communication.
Example Answers
In my previous role, I identified discrepancies in customer data that affected billing accuracy. I organized a meeting with the sales team, using clear visuals to illustrate the impact on revenue. I explained how these errors could affect customer trust. Together, we outlined steps to improve data entry processes, resulting in a 20% reduction in errors.
Describe a time you received constructive criticism about your work in data quality. How did you respond?
How to Answer
- 1
Choose a specific example from your experience.
- 2
Be honest about the criticism received.
- 3
Explain how you reflected on the feedback.
- 4
Describe the actions you took to improve.
- 5
Share the positive outcome or results of your response.
Example Answers
In my previous role, I was told my data validation process was too slow. I took the feedback seriously, examined my approach, and streamlined the validation steps, which improved efficiency by 30%.
What do you consider your greatest achievement in your data quality career and why?
How to Answer
- 1
Choose a specific project or initiative that had measurable impact
- 2
Highlight your role and the skills you used to achieve success
- 3
Include quantitative results to demonstrate effectiveness
- 4
Explain the significance of the achievement for the organization
- 5
Mention any recognition or feedback you received from stakeholders
Example Answers
My greatest achievement was leading a data cleansing project that improved data accuracy by 30%. I spearheaded the initiative, using advanced tools to identify discrepancies. The enhanced data quality contributed to better decision-making and was well-received by upper management.
Don't Just Read Data Quality Analyst Questions - Practice Answering Them!
Reading helps, but actual practice is what gets you hired. Our AI feedback system helps you improve your Data Quality Analyst interview answers in real-time.
Personalized feedback
Unlimited practice
Used by hundreds of successful candidates
Technical Interview Questions
What tools and methods do you typically use to assess data quality?
How to Answer
- 1
Mention specific tools like Python libraries (Pandas, Dask) or SQL for data profiling.
- 2
Discuss methods such as data validation, data cleansing, and anomaly detection.
- 3
Highlight the importance of defining data quality metrics like accuracy, completeness, and consistency.
- 4
Emphasize collaboration with stakeholders to understand their data quality requirements.
- 5
Provide examples of how you've implemented these tools and methods in past roles.
Example Answers
I typically use Python libraries like Pandas for data profiling and SQL queries to validate data against predefined rules. I focus on metrics like accuracy and completeness to ensure high data quality.
Can you explain the difference between data validation and data verification?
How to Answer
- 1
Define each term briefly to show understanding.
- 2
Highlight the purpose of data validation.
- 3
Emphasize the purpose of data verification.
- 4
Mention typical methods or techniques used in each process.
- 5
Provide an example to illustrate the differences.
Example Answers
Data validation checks if the data is correct and appropriate for its intended use, like ensuring a date field contains a valid date. Data verification confirms that the data is accurate and matches the source, like comparing entries against a master list.
Don't Just Read Data Quality Analyst Questions - Practice Answering Them!
Reading helps, but actual practice is what gets you hired. Our AI feedback system helps you improve your Data Quality Analyst interview answers in real-time.
Personalized feedback
Unlimited practice
Used by hundreds of successful candidates
What is data profiling, and why is it important in data quality management?
How to Answer
- 1
Define data profiling clearly as the process of examining data for completeness, accuracy, and quality.
- 2
Highlight its role in identifying data quality issues early in the data lifecycle.
- 3
Mention how it helps in understanding data characteristics, such as patterns and distributions.
- 4
Explain its importance in supporting data governance and regulatory compliance.
- 5
Emphasize its utility in informing decisions for data cleaning and transformation efforts.
Example Answers
Data profiling is the process of analyzing data to assess its quality and structure. It is vital because it uncovers issues early, allowing teams to address them before they escalate. This helps ensure data integrity, supports compliance, and guides decisions for effective data management.
What programming languages or query languages are you proficient in for data analysis?
How to Answer
- 1
List the languages you know, focusing on those relevant to data analysis
- 2
Mention your experience level for each language, e.g., beginner, intermediate, advanced
- 3
Provide examples of projects or tasks where you used these languages
- 4
Highlight any specific libraries or tools associated with the languages
- 5
Be prepared to discuss how these languages have aided in ensuring data quality
Example Answers
I am proficient in SQL and Python, with intermediate experience in both. I've used SQL for data extraction and transformation in various projects, and Python for data cleaning using libraries like Pandas and NumPy.
What experience do you have with data quality tools, such as Talend, Informatica, or others?
How to Answer
- 1
Identify specific tools you've used and your level of experience
- 2
Mention any projects where you applied these tools to improve data quality
- 3
Highlight any certifications or training related to these tools
- 4
Discuss your familiarity with data profiling, cleansing, and validation processes
- 5
Emphasize outcomes or improvements resulting from your work with these tools
Example Answers
I have used Talend extensively for data integration and quality. In my last project, I implemented Talend to automate data cleansing, which reduced errors by 30%.
What is your understanding of ETL processes, and how do they relate to data quality?
How to Answer
- 1
Define ETL: Explain Extraction, Transformation, and Loading clearly.
- 2
Highlight the importance of data quality at each ETL stage.
- 3
Mention common data quality issues like duplicates, inaccuracies, and completeness.
- 4
Discuss how proper ETL processes can improve data quality.
- 5
Provide examples of tools or techniques used to enhance data quality during ETL.
Example Answers
ETL stands for Extraction, Transformation, and Loading. During the extraction phase, data is pulled from various sources. In transformation, we clean and enrich the data to ensure accuracy and consistency. Finally, loading involves placing the data into a target system. Each ETL stage is crucial for maintaining high data quality, addressing issues like duplicates and inaccuracies, ensuring that we work with reliable data.
What key performance indicators (KPIs) do you use to measure data quality?
How to Answer
- 1
List specific KPIs that are relevant to data quality such as accuracy, completeness, consistency, timeliness, and uniqueness.
- 2
Explain why each KPI is important for data quality evaluation.
- 3
Provide examples of how you have measured these KPIs in previous roles.
- 4
Mention any tools or methods you used for tracking these KPIs.
- 5
Highlight the impact of good data quality on business decisions.
Example Answers
I measure data quality using accuracy, completeness, and consistency. For instance, I validate accuracy by cross-referencing data against reliable sources. Completeness is checked by ensuring all required fields are filled, and consistency is verified using data profiling tools. These KPIs help ensure that stakeholders can trust the data for decision-making.
What role do you think data governance plays in ensuring data quality?
How to Answer
- 1
Emphasize the definition of data governance and its importance.
- 2
Discuss how data governance sets standards for data quality.
- 3
Highlight the accountability established through governance frameworks.
- 4
Mention the role of data stewardship in maintaining quality.
- 5
Explain how governance supports compliance and reduces risk.
Example Answers
Data governance provides a framework for maintaining data quality by establishing consistent standards and accountability. It ensures that data is accurate and reliable, which is essential for decision-making.
What strategies do you employ for data cleansing and making datasets usable?
How to Answer
- 1
Identify and remove duplicates using tools like Python or SQL.
- 2
Standardize formats for dates, names, and other key fields.
- 3
Utilize automated scripts for common cleansing tasks to save time.
- 4
Conduct thorough validation checks to verify data accuracy post-cleansing.
- 5
Document the cleansing process to ensure transparency and reproducibility.
Example Answers
I start by identifying duplicates through SQL queries and remove them. Then, I standardize date formats throughout the dataset. I often write automated scripts for repetitive tasks, ensuring consistency.
How would you visualize data quality metrics to communicate findings effectively?
How to Answer
- 1
Identify key metrics that reflect data quality.
- 2
Use visualizations like bar charts for comparison and pie charts for proportions.
- 3
Incorporate dashboards for real-time data monitoring.
- 4
Utilize colors to indicate data quality levels, such as red for poor quality and green for good.
- 5
Explain insights clearly alongside visuals to provide context.
Example Answers
I would focus on metrics like accuracy, completeness, and consistency, using bar charts to compare these metrics across different datasets. I would also create a dashboard with real-time updates and color coding to quickly highlight areas needing attention.
Don't Just Read Data Quality Analyst Questions - Practice Answering Them!
Reading helps, but actual practice is what gets you hired. Our AI feedback system helps you improve your Data Quality Analyst interview answers in real-time.
Personalized feedback
Unlimited practice
Used by hundreds of successful candidates
How do you ensure data quality when working with third-party data sources?
How to Answer
- 1
Conduct a preliminary assessment of the third-party source for credibility and reliability
- 2
Implement data validation rules to check for accuracy and consistency upon ingestion
- 3
Regularly monitor and audit the data for anomalies and discrepancies
- 4
Establish clear communication with third-party providers for data updates and issues
- 5
Document data quality metrics and processes for ongoing improvement
Example Answers
I begin by assessing the third-party source to confirm its credibility. Upon receiving data, I apply validation rules to ensure its accuracy. I also conduct regular audits to spot any discrepancies, allowing me to address issues promptly.
Situational Interview Questions
If a stakeholder is unsatisfied with the data quality reports you produce, how would you handle the situation?
How to Answer
- 1
Listen to the stakeholder's concerns carefully and ask clarifying questions
- 2
Acknowledge their dissatisfaction and express a willingness to improve
- 3
Review the reports in question to identify potential gaps or misunderstandings
- 4
Offer to collaborate on a revised report that better meets their needs
- 5
Follow up after implementation to ensure their expectations are met
Example Answers
I would first listen to the stakeholder's concerns to understand their specific issues. Then, I would thank them for their feedback and assure them that I'm committed to improving the reports. I would review the reports to identify any gaps and suggest working together to create a revised version that addresses their needs.
Imagine you discover a critical data integrity issue just before a reporting deadline. What steps would you take?
How to Answer
- 1
Quickly assess the severity and impact of the data issue
- 2
Communicate with stakeholders to inform them of the problem
- 3
Investigate the root cause and data source of the issue
- 4
Implement a temporary solution if possible to meet the deadline
- 5
Document the issue and follow up with a plan for permanent resolution
Example Answers
I would first evaluate how severe the data integrity issue is and what reports it affects. Then, I would notify the relevant stakeholders immediately so they are aware of potential impacts. Next, I'd dig into the data sources to identify the root cause and take steps to address it. If feasible, I would apply a temporary fix to ensure we meet the reporting deadline while planning for a comprehensive solution afterward.
Don't Just Read Data Quality Analyst Questions - Practice Answering Them!
Reading helps, but actual practice is what gets you hired. Our AI feedback system helps you improve your Data Quality Analyst interview answers in real-time.
Personalized feedback
Unlimited practice
Used by hundreds of successful candidates
You have multiple data quality issues to address, each with high importance. How would you prioritize them?
How to Answer
- 1
Assess the impact of each issue on business operations.
- 2
Determine the frequency and severity of the issues.
- 3
Consider stakeholder needs and urgency.
- 4
Evaluate the resources required to resolve each issue.
- 5
Aim for quick wins to build momentum while addressing bigger challenges.
Example Answers
I would first evaluate which data quality issues have the most significant impact on critical business functions. Then, I'd prioritize based on frequency and severity, ensuring that stakeholders' urgent needs are met.
How would you approach a scenario where the cause of poor data quality is unclear or complex?
How to Answer
- 1
Start by gathering all available data and documentation related to the issue.
- 2
Conduct interviews with key stakeholders to understand their perspectives and insights.
- 3
Use data profiling techniques to analyze the data for patterns or anomalies.
- 4
Develop a hypothesis and test it by isolating variables in the data.
- 5
Implement a feedback loop to continuously refine your approach based on findings.
Example Answers
I would first collect all relevant data and documentation, and then speak with stakeholders to gather their input. Next, I would analyze the data through profiling techniques to uncover patterns. By forming a hypothesis and testing it, I could identify the root cause of the poor data quality.
If a key stakeholder refuses to adopt the data quality measures you recommend, what would you do?
How to Answer
- 1
Listen to their concerns and understand their perspective
- 2
Present data and case studies that illustrate the benefits of the measures
- 3
Engage in a collaborative discussion to identify their priorities
- 4
Offer compromises or alternatives that align with their goals
- 5
Follow up regularly to build trust and show progress over time
Example Answers
I would start by listening to the stakeholder's concerns to understand their perspective. Then, I'd present relevant data and case studies that demonstrate the benefits of the proposed measures, aiming to engage them in a collaborative discussion to find common ground.
If a recurring data quality issue is reported, how would you conduct a root cause analysis?
How to Answer
- 1
Gather detailed information about the issue from stakeholders and users
- 2
Analyze the data sets involved to identify patterns or anomalies
- 3
Check the data flow and transformation processes for errors
- 4
Consult logging and monitoring tools for any relevant error reports
- 5
Document findings and suggest actionable solutions based on root cause
Example Answers
I would start by collecting reports from users experiencing the issue, then analyze the datasets for unusual patterns. Next, I would review the data flow and transformation steps, and check any relevant logs for errors. Finally, I would document the findings and propose targeted solutions to prevent recurrence.
How would you handle a situation where you have tight deadlines to improve data quality and limited resources?
How to Answer
- 1
Prioritize the most impactful data quality issues first
- 2
Leverage existing tools and automation to save time
- 3
Engage stakeholders to align on critical data needs
- 4
Document processes to streamline future quality checks
- 5
Communicate clearly about limitations and progress
Example Answers
I would start by identifying the top 3 data quality issues that have the greatest impact on decision-making. Then, I'd use available data quality tools to automate checks and corrections where possible. Engaging with stakeholders would ensure we focus on what matters most, and I'd document our approach for future reference.
You are assigned to a project to improve the quality of a dataset that has been neglected. What is your action plan?
How to Answer
- 1
Assess the current state of the dataset to identify key quality issues
- 2
Develop a data quality framework including accuracy, completeness, consistency, and timeliness
- 3
Engage stakeholders to understand their requirements and expectations
- 4
Implement data cleansing processes to fix errors and standardize formats
- 5
Establish ongoing monitoring and validation procedures to maintain quality
Example Answers
First, I would conduct a thorough assessment of the dataset to identify prevalent issues such as missing values and duplicates. Then, I would create a quality framework to address those issues. Engaging with stakeholders will help clarify their needs, and subsequently, I would initiate data cleaning methods to rectify the identified problems. Finally, I would set up a monitoring system to ensure continuing data quality.
How would you approach implementing a new data quality process in your team?
How to Answer
- 1
Assess the current data quality issues and sources of error
- 2
Engage team members to gather input and foster collaboration
- 3
Define clear metrics for data quality success and standards
- 4
Develop a step-by-step implementation plan with timelines
- 5
Provide training and resources to support the team during the transition
Example Answers
I would start by evaluating our existing data to identify common quality issues. Then, I would involve the team to collect their insights and suggestions. Once we have a clear understanding, I would establish specific data quality metrics to track our progress and create an actionable plan for implementation. Training sessions would be arranged to ensure everyone understands the new processes.
Data Quality Analyst Position Details
Salary Information
Recommended Job Boards
CareerBuilder
www.careerbuilder.com/jobs/data-quality-analystZipRecruiter
www.ziprecruiter.com/Jobs/Data-Quality-AnalystThese job boards are ranked by relevance for this position.
Related Positions
Ace Your Next Interview!
Practice with AI feedback & get hired faster
Personalized feedback
Used by hundreds of successful candidates
Ace Your Next Interview!
Practice with AI feedback & get hired faster
Personalized feedback
Used by hundreds of successful candidates