Top 30 Marketing Data Scientist Interview Questions and Answers [Updated 2025]
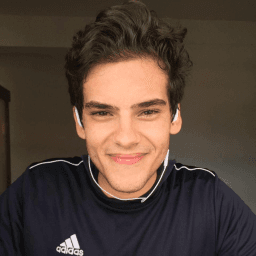
Andre Mendes
•
March 30, 2025
Are you preparing for a Marketing Data Scientist interview and eager to make a lasting impression? This blog post is your ultimate guide to acing that interview, featuring the most common and insightful questions tailored for this dynamic role. Discover example answers and expert tips on how to respond effectively, equipping you with the confidence and knowledge to stand out from the competition. Dive in and elevate your interview game!
Download Marketing Data Scientist Interview Questions in PDF
To make your preparation even more convenient, we've compiled all these top Marketing Data Scientistinterview questions and answers into a handy PDF.
Click the button below to download the PDF and have easy access to these essential questions anytime, anywhere:
List of Marketing Data Scientist Interview Questions
Behavioral Interview Questions
Describe a time when you had to work closely with a marketing team to understand their needs and deliver actionable insights.
How to Answer
- 1
Identify a specific project where you collaborated with marketing.
- 2
Explain the marketing team's objectives and how you addressed them.
- 3
Discuss the data analysis methods you used to generate insights.
- 4
Highlight the actionable recommendations you provided based on your findings.
- 5
Describe the outcome and how it benefited the marketing team.
Example Answers
In my previous role, I worked on a campaign analysis project with the marketing team. They needed to understand the effectiveness of different channels. I analyzed campaign data and used A/B testing results, which led to insights that the email channel was underutilized. As a result, we reallocated budget and increased engagement by 25%.
Tell me about a challenging data problem you faced in your previous job and how you resolved it.
How to Answer
- 1
Identify a specific data problem you encountered.
- 2
Explain the context and challenges briefly.
- 3
Outline the steps you took to analyze and solve the problem.
- 4
Mention any tools and techniques you used.
- 5
Conclude with the outcome and what you learned.
Example Answers
In my previous role, we had inaccurate customer segmentation data which affected targeting. I analyzed the data using Python and identified inconsistencies. I cleaned the data and applied clustering techniques, which improved our segmentation accuracy by 30%. We used this to refine our marketing campaigns, leading to a 15% increase in conversion rates.
Don't Just Read Marketing Data Scientist Questions - Practice Answering Them!
Reading helps, but actual practice is what gets you hired. Our AI feedback system helps you improve your Marketing Data Scientist interview answers in real-time.
Personalized feedback
Unlimited practice
Used by hundreds of successful candidates
Give an example of how you have communicated complex data findings to a non-technical audience.
How to Answer
- 1
Identify the key findings to share
- 2
Use visuals like charts to simplify data
- 3
Avoid jargon and technical terms
- 4
Relate findings to the audience's interests
- 5
Tell a story to make data relatable
Example Answers
In my last project, I analyzed customer behavior data and found that younger customers preferred mobile shopping. I created a simple infographic that highlighted this trend using clear visuals, and I explained how this could influence our marketing strategy, avoiding technical terms to ensure everyone understood.
Can you describe a time when you had to adapt to significant changes in a project or dataset?
How to Answer
- 1
Identify a specific project where changes occurred
- 2
Explain what the changes were and why they happened
- 3
Describe the steps you took to adapt to the changes
- 4
Highlight the outcome or results from your adaptation
- 5
Reflect on what you learned from the experience
Example Answers
In my last project, we had to switch datasets due to data quality issues. I quickly researched alternative sources and implemented a new data cleaning process. This adaptation led to improved accuracy in our model, and the stakeholders were pleased with the timely delivery.
Have you ever led a data science project? What was your approach and outcome?
How to Answer
- 1
Define the project's goal clearly and concisely.
- 2
Explain the steps taken in the project lifecycle.
- 3
Highlight specific tools and methodologies used.
- 4
Discuss team collaboration or leadership aspects.
- 5
Summarize the results or impact of the project.
Example Answers
I led a project aimed at improving customer retention. We used machine learning models to analyze customer behavior. I guided the team through data cleaning, feature engineering, and model selection using Python. We collaborated closely with the marketing team to implement our findings, resulting in a 15% increase in retention rates.
Describe a situation where you had a conflict with a team member about data analysis approaches. How was it resolved?
How to Answer
- 1
Identify the key conflict and analysis methods being debated
- 2
Describe your perspective and reasoning behind your approach
- 3
Explain how you listened to your team member’s viewpoint
- 4
Highlight collaboration or compromise that led to a solution
- 5
Conclude with the outcome and what you learned from the experience
Example Answers
In a recent project, I disagreed with a colleague on whether to use a logistic regression model or a decision tree for our predictions. I explained that regression would give us clearer coefficients for interpretation, but she argued for decision trees due to their flexibility. We decided to run both models and compared results, which showed that the decision tree performed better due to the complexity of the dataset. This taught us the value of testing multiple approaches.
Can you give an example of a time you proactively identified a business opportunity through data?
How to Answer
- 1
Choose a specific project or analysis you worked on
- 2
Explain the data sources you used and how you analyzed them
- 3
Describe the business opportunity you identified
- 4
Discuss the impact of your findings on the business
- 5
Use quantitative results to support your example
Example Answers
In my last role, I analyzed customer purchase data and noticed that a significant segment was repeatedly purchasing outdoor gear. I suggested launching a targeted marketing campaign for this segment, which increased sales by 30% in that category over three months.
How do you prioritize your tasks when handling multiple data science projects?
How to Answer
- 1
Identify project deadlines and strategic importance to the business
- 2
Break down tasks into manageable components and estimate time required
- 3
Communicate with stakeholders to understand their priorities and expectations
- 4
Use a project management tool to visualize progress and prioritize tasks
- 5
Regularly reassess priorities as new information and projects arrive
Example Answers
I start by assessing the deadlines and business impact of each project. Then, I break down the projects into tasks and estimate how long each will take. I keep open communication with stakeholders to ensure I'm aligned with their needs. I also use a project management tool to track my progress and adjust my priorities as needed.
Describe how you manage your time when working on a project with a tight deadline.
How to Answer
- 1
Break the project into smaller tasks to manage them more easily
- 2
Set priorities based on urgency and impact to focus on what matters
- 3
Use time blocking to allocate specific periods for each task
- 4
Regularly review progress and adjust your plan if needed
- 5
Communicate with your team to ensure alignment on goals and deadlines
Example Answers
When facing a tight deadline, I break the project into smaller tasks and prioritize them by urgency and importance. I then block off specific time slots in my calendar to work on each task and check in with my team regularly to ensure we're all on the same page.
Describe a project where you introduced an innovative data analysis approach. What was the impact?
How to Answer
- 1
Select a project with measurable outcomes.
- 2
Explain the innovative approach you used succinctly.
- 3
Highlight the problem you solved with this approach.
- 4
Include specific metrics or results to demonstrate impact.
- 5
Discuss any feedback or recognition received.
Example Answers
In a recent project, I implemented a machine learning forecast model to predict customer churn. This innovative approach reduced churn by 15%, resulting in $200,000 in increased revenue over six months. The team praised the model for its accuracy.
Don't Just Read Marketing Data Scientist Questions - Practice Answering Them!
Reading helps, but actual practice is what gets you hired. Our AI feedback system helps you improve your Marketing Data Scientist interview answers in real-time.
Personalized feedback
Unlimited practice
Used by hundreds of successful candidates
Technical Interview Questions
What machine learning algorithms are you most familiar with and how have you applied them in a marketing context?
How to Answer
- 1
Identify 2-3 machine learning algorithms you know well.
- 2
Briefly describe a specific project where you used each algorithm.
- 3
Highlight the marketing outcomes or insights gained from your application.
- 4
Use metrics or results to demonstrate the effectiveness of your approach.
- 5
Keep your explanation clear and relevant to marketing data science.
Example Answers
I am familiar with logistic regression and decision trees. In a recent campaign analysis, I used logistic regression to predict customer churn, which helped us reduce churn rates by 15%.
How do you ensure the accuracy and reliability of the data you work with?
How to Answer
- 1
Perform data validation checks and outlier analysis regularly.
- 2
Cross-check data sources to ensure consistency.
- 3
Utilize automated tools to monitor data quality.
- 4
Document data cleaning steps and transformations.
- 5
Engage with stakeholders to clarify data needs and requirements.
Example Answers
I ensure data accuracy by implementing validation checks at the collection stage and regularly running outlier analysis to catch anomalies early.
Don't Just Read Marketing Data Scientist Questions - Practice Answering Them!
Reading helps, but actual practice is what gets you hired. Our AI feedback system helps you improve your Marketing Data Scientist interview answers in real-time.
Personalized feedback
Unlimited practice
Used by hundreds of successful candidates
What programming languages do you use for data analysis and why?
How to Answer
- 1
Identify the key programming languages you are proficient in.
- 2
Explain each language's strengths in data analysis.
- 3
Mention any libraries or tools relevant to those languages.
- 4
Relate your experience with real-world applications or projects.
- 5
Tailor your response to the job description if possible.
Example Answers
I primarily use Python because of its rich libraries like Pandas and NumPy for data manipulation and analysis. It's versatile and widely used in data science projects.
What tools do you use for data visualization, and how do you choose which ones to use?
How to Answer
- 1
Identify popular tools you use like Tableau or Power BI.
- 2
Explain your selection criteria based on the project needs.
- 3
Mention the importance of audience and data type in your choice.
- 4
Give an example of a visualization project you worked on.
- 5
Highlight any collaboration with stakeholders for input on the tools used.
Example Answers
I primarily use Tableau and Power BI for data visualization because they allow for interactive dashboards. I choose tools based on the complexity of the data and the specific needs of the audience. For instance, I used Tableau for a project visualizing customer trends as it provided the necessary interactivity for stakeholders.
What statistical methods do you use to analyze marketing data, and how do you decide which ones to apply?
How to Answer
- 1
Identify specific statistical methods that are relevant to marketing, like regression analysis, A/B testing, or clustering.
- 2
Explain the context in which you would use each method based on the data and the goals of the analysis.
- 3
Discuss how data characteristics such as distribution and sample size influence your choice of methods.
- 4
Mention any tools or software you prefer for statistical analysis and why.
- 5
Provide examples of past projects where you applied these methods effectively.
Example Answers
I frequently use regression analysis to predict customer lifetime value from past purchase behavior. For experimental data, I rely on A/B testing to evaluate marketing campaign effectiveness, adapting my choice based on sample size and the nature of the expected results.
How do you handle and analyze large datasets in your work?
How to Answer
- 1
Use efficient data processing libraries like pandas for Python or data.table for R.
- 2
Break down large datasets into manageable chunks whenever possible for analysis.
- 3
Utilize SQL for querying and filtering large datasets, ensuring optimized performance.
- 4
Leverage cloud computing resources for scalable storage and processing on large datasets.
- 5
Visualize data using tools like Tableau or Matplotlib to derive insights quickly.
Example Answers
I typically use pandas to handle large datasets, slicing them into smaller pieces for manageable analysis. I also use SQL to extract relevant data before loading into Python for deeper exploration.
What experience do you have with building or managing ETL processes?
How to Answer
- 1
Mention specific tools you used, like Apache Airflow, Talend, or custom Python scripts.
- 2
Describe a specific ETL project you worked on, focusing on your role.
- 3
Highlight how you ensured data quality and handled errors.
- 4
Discuss the volume of data you processed and how you optimized the process.
- 5
Explain how you collaborated with team members or stakeholders during the project.
Example Answers
I built an ETL process using Apache Airflow for a marketing analytics project. My role involved designing the data pipeline, which extracted data from various sources, transformed it using Python, and loaded it into our data warehouse. I implemented error handling to ensure data quality and collaborated closely with our data engineers.
What are your favorite Python libraries for data science, and how do you use them?
How to Answer
- 1
Identify 3 to 5 libraries you use regularly.
- 2
Explain specific use cases for each library.
- 3
Mention any personal projects where you utilized these libraries.
- 4
Highlight any advanced features or functions you appreciate.
- 5
Be prepared to discuss how these libraries help in data analysis and modeling.
Example Answers
I love using pandas for data manipulation, especially for cleaning and transforming datasets. I often use it in my personal projects for exploratory data analysis, combined with NumPy for numerical computations.
What strategies do you use for data cleaning and preprocessing?
How to Answer
- 1
Identify missing values and decide whether to fill or remove them.
- 2
Standardize data formats and units for consistency.
- 3
Remove duplicates to ensure data integrity.
- 4
Use outlier detection methods to clean anomalies from the dataset.
- 5
Transform categorical variables into numerical for analysis.
Example Answers
I start by checking for missing values and either fill them using methods like mean or median imputation, or I remove the rows if they have too many NAs. Then, I standardize date formats and units across the dataset to ensure consistency.
Can you write an SQL query to calculate the average order value from a sales database?
How to Answer
- 1
Identify the relevant tables that contain sales data.
- 2
Determine the correct columns for order total and order count.
- 3
Use the AVG() function to calculate the average.
- 4
Ensure to group by any necessary fields if required.
- 5
Review the query syntax for accuracy before presenting.
Example Answers
SELECT AVG(order_total) AS average_order_value FROM sales;
Don't Just Read Marketing Data Scientist Questions - Practice Answering Them!
Reading helps, but actual practice is what gets you hired. Our AI feedback system helps you improve your Marketing Data Scientist interview answers in real-time.
Personalized feedback
Unlimited practice
Used by hundreds of successful candidates
Situational Interview Questions
Imagine you have data that shows a drop in customer engagement for a marketing campaign. How would you approach analyzing this?
How to Answer
- 1
Identify key metrics to analyze engagement over time.
- 2
Segment the customer data to understand which groups are affected.
- 3
Investigate changes in marketing tactics or external factors during the drop.
- 4
Use visualizations to identify trends and anomalies in the data.
- 5
Develop hypotheses to test why engagement dropped and explore potential solutions.
Example Answers
I would start by looking at key engagement metrics like click-through rates and conversion rates over the campaign period. Then, I would segment the data by demographics to see if a specific group is underperforming.
You are tasked with evaluating the success of a marketing campaign using data. What metrics would you prioritize and why?
How to Answer
- 1
Identify key performance indicators relevant to the campaign's goals
- 2
Consider both immediate metrics and long-term impact metrics
- 3
Explain how each metric relates to campaign success
- 4
Use specific data points to support your argument
- 5
Relate metrics to actionable insights for future campaigns
Example Answers
I would prioritize conversion rate and return on investment (ROI) as they indicate how effective the campaign is in driving sales and revenue. Additionally, tracking customer acquisition cost helps evaluate the efficiency of marketing spend.
Don't Just Read Marketing Data Scientist Questions - Practice Answering Them!
Reading helps, but actual practice is what gets you hired. Our AI feedback system helps you improve your Marketing Data Scientist interview answers in real-time.
Personalized feedback
Unlimited practice
Used by hundreds of successful candidates
If you were to conduct an A/B test for a new product feature, how would you design the experiment?
How to Answer
- 1
Define the objective of the A/B test clearly.
- 2
Identify the key metrics to measure success.
- 3
Decide on the sample size and randomization method.
- 4
Set up the control and experimental groups.
- 5
Plan the duration of the test and ensure statistical validity.
Example Answers
First, I would define the objective, like increasing user engagement. Next, I'll track metrics such as click-through rate. I'll use random sampling to split users into control and experimental groups. Finally, I would run the test for two weeks to ensure reliable results.
How would you handle a situation where marketing stakeholders disagree with your data-driven recommendations?
How to Answer
- 1
Listen actively to the stakeholders' concerns and viewpoints.
- 2
Present your data and findings clearly and concisely.
- 3
Use visual aids to help illustrate your points if applicable.
- 4
Encourage an open discussion to explore the stakeholders' arguments further.
- 5
Suggest a compromise or a pilot test to validate your recommendations.
Example Answers
I would first listen to the stakeholders' concerns to understand their perspective. Then, I'd present my data clearly and use visuals to illustrate key points. Encouraging discussion allows us to explore their arguments, and I might suggest a pilot test to validate my recommendations.
You need to forecast sales for the next quarter using historical data. How would you go about this?
How to Answer
- 1
Gather and clean the historical sales data for at least the last few quarters.
- 2
Analyze trends and seasonality in the data using time series analysis.
- 3
Choose a forecasting method such as ARIMA, exponential smoothing, or machine learning models.
- 4
Split the data into a training set and a test set to validate your model.
- 5
Evaluate the accuracy of your forecast and adjust your model as necessary.
Example Answers
First, I would collect historical sales data from the last two years and clean it to remove outliers. Then, I'd analyze the data for trends and seasonal patterns using a time series decomposition. Next, I would implement an ARIMA model to create the forecast and validate its accuracy using a test set.
If you were asked to evaluate the ROI of several marketing channels, how would you proceed?
How to Answer
- 1
Identify the marketing channels to evaluate
- 2
Gather data on costs associated with each channel
- 3
Collect performance metrics such as leads or sales generated
- 4
Calculate ROI using the formula: ROI = (Net Profit / Cost) * 100
- 5
Compare the ROIs to determine the most effective channels
Example Answers
First, I would list all marketing channels such as social media, email, and PPC, then gather data on the costs for each channel. I would analyze their performance in terms of leads or sales generated. Using the ROI formula, I would calculate the ROI for each channel and compare them to identify which channels provide the best return.
How would you respond if a stakeholder asks you to manipulate data in a way that seems unethical?
How to Answer
- 1
Acknowledge the request calmly and professionally
- 2
Explain the importance of ethical data practices
- 3
Provide alternatives that uphold data integrity
- 4
Offer to collaborate on finding a solution that meets their needs
- 5
Document the conversation to keep a record of the request
Example Answers
I would first acknowledge the stakeholder's request and ask for clarification on their objectives. Then, I would explain why the proposed manipulation is unethical, emphasizing that integrity is crucial. I would suggest alternative ways to analyze or present the data that maintain ethical standards.
How would you approach building a customer segmentation model for a marketing team?
How to Answer
- 1
Define the objective of the segmentation clearly.
- 2
Gather and preprocess relevant data from various sources.
- 3
Choose appropriate segmentation techniques like k-means or hierarchical clustering.
- 4
Evaluate and validate segments using metrics like silhouette score.
- 5
Communicate findings to the marketing team with actionable insights.
Example Answers
To build a customer segmentation model, I would first clarify the goal, such as increasing campaign effectiveness. Then, I would collect data on customer demographics and behaviors, followed by preprocessing the data. Next, I would use k-means clustering to segment customers, evaluate the segments using silhouette score, and finally present these insights to the marketing team to inform their strategies.
You need to collaborate with the IT department to get data from a new database. How would you facilitate this process?
How to Answer
- 1
Initiate a meeting with IT to discuss project requirements
- 2
Clearly outline the data needed and its intended use
- 3
Ensure you understand their process and requirements
- 4
Be open to feedback and flexibility in your requests
- 5
Follow up with documentation summarizing the agreements made
Example Answers
I would start by setting up a meeting with the IT department to explain what data I need and how it will benefit our marketing efforts. I would make sure to listen to their concerns and requirements in the process.
You are tasked with designing a new experiment to test a marketing hypothesis. What steps would you take?
How to Answer
- 1
Define a clear marketing hypothesis to test.
- 2
Determine the key metrics for success and how to measure them.
- 3
Choose an appropriate experimental design, such as A/B testing.
- 4
Identify the target audience and how to randomly assign them.
- 5
Plan for data collection and analysis post-experiment.
Example Answers
First, I would clearly define my marketing hypothesis, like 'offering a discount increases conversion rates.' Then, I'd identify key metrics, such as conversion rate and average order value. Next, I would set up an A/B test with a control group and a treatment group. After that, I'd choose my target audience randomly to ensure unbiased results, and finally, I'd plan how to collect and analyze the data once the experiment runs.
Don't Just Read Marketing Data Scientist Questions - Practice Answering Them!
Reading helps, but actual practice is what gets you hired. Our AI feedback system helps you improve your Marketing Data Scientist interview answers in real-time.
Personalized feedback
Unlimited practice
Used by hundreds of successful candidates
Marketing Data Scientist Position Details
Salary Information
Average Salary
$100,874
Source: PayScale
Recommended Job Boards
CareerBuilder
www.careerbuilder.com/jobs/marketing-data-scientistThese job boards are ranked by relevance for this position.
Related Positions
Ace Your Next Interview!
Practice with AI feedback & get hired faster
Personalized feedback
Used by hundreds of successful candidates
Ace Your Next Interview!
Practice with AI feedback & get hired faster
Personalized feedback
Used by hundreds of successful candidates