Top 30 Analytical Statistician Interview Questions and Answers [Updated 2025]
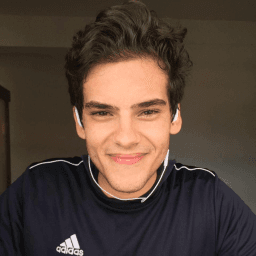
Andre Mendes
•
March 30, 2025
Navigating the competitive landscape of analytical statistician interviews can be daunting, but mastering the most common questions can give you a significant edge. In this updated guide for 2025, we provide a comprehensive list of essential interview questions, complete with example answers and insightful tips to help you respond with confidence and clarity. Prepare to elevate your interview skills and make a lasting impression.
Download Analytical Statistician Interview Questions in PDF
To make your preparation even more convenient, we've compiled all these top Analytical Statisticianinterview questions and answers into a handy PDF.
Click the button below to download the PDF and have easy access to these essential questions anytime, anywhere:
List of Analytical Statistician Interview Questions
Behavioral Interview Questions
Describe a time when you worked in a team to analyze a complex dataset. What was your role and how did you contribute to the team’s success?
How to Answer
- 1
Select a specific project where teamwork was essential.
- 2
Clearly define your role and responsibilities within the team.
- 3
Emphasize your analytical skills and techniques used.
- 4
Discuss the outcome and how the team's efforts led to success.
- 5
Reflect on what you learned from the experience.
Example Answers
In my last internship, I worked on a team analyzing customer survey data. My role was to clean and preprocess the data using Python. I then conducted statistical analyses and shared insights during our meetings. As a result, our findings helped the marketing team improve their campaigns, leading to a 20% increase in engagement.
Tell me about a challenging analytical problem you solved. What made it challenging and what was your approach to solving it?
How to Answer
- 1
Choose a specific real problem you've faced in your work
- 2
Explain the complexity of the problem clearly
- 3
Outline your thought process and the methods you used
- 4
Highlight any collaboration or resources that were important
- 5
Conclude with the outcome and what you learned
Example Answers
In my previous job, I had to analyze a dataset with missing values and outliers that were skewing the results. The complexity arose from the need to maintain the integrity of the data while ensuring reliable conclusions. I used multiple imputation techniques and robust statistical methods to address the outliers. I collaborated with a data engineer to clean the data effectively, which helped me achieve more accurate results. Ultimately, the analysis informed a key decision that improved our marketing strategy.
Don't Just Read Analytical Statistician Questions - Practice Answering Them!
Reading helps, but actual practice is what gets you hired. Our AI feedback system helps you improve your Analytical Statistician interview answers in real-time.
Personalized feedback
Unlimited practice
Used by hundreds of successful candidates
Can you provide an example of how you explained a complex statistical concept to a non-technical audience?
How to Answer
- 1
Choose a specific statistical concept you've explained.
- 2
Describe the audience and why they needed the explanation.
- 3
Use relatable examples or analogies to simplify the concept.
- 4
Keep technical jargon minimal to avoid confusion.
- 5
Summarize how the audience responded or what they learned.
Example Answers
I explained the concept of regression analysis to a group of marketing managers. I compared it to predicting sales based on the weather, showing how different factors like temperature and promotions interact. I used a simple graph to illustrate trends, and by the end, they felt confident discussing how different variables affect sales.
Describe a situation where you had a disagreement with a colleague over a data analysis approach. How was it resolved?
How to Answer
- 1
Focus on a specific example that illustrates the disagreement.
- 2
Clearly explain your stance and the rationale behind it.
- 3
Discuss how you communicated with your colleague about the differing views.
- 4
Highlight the resolution process, emphasizing collaboration.
- 5
Conclude with the outcome and any lessons learned.
Example Answers
In a project, I disagreed with a colleague who wanted to use linear regression for prediction, while I suggested decision trees. I explained that the data was non-linear. We had a discussion and decided to test both models. Ultimately, the decision tree performed better, and we combined our methods for a more robust solution.
Give an example of how you managed a project with tight deadlines and multiple priorities in your analytical work.
How to Answer
- 1
Identify specific projects you managed under similar conditions
- 2
Discuss prioritization strategies you used
- 3
Mention tools or methods that helped you stay organized
- 4
Highlight teamwork and communication efforts
- 5
Reflect on the outcome and what you learned
Example Answers
In my previous role, I was tasked with analyzing customer data for a marketing campaign while also preparing a quarterly report. I prioritized the campaign data since the deadline was closer, and I used a project management tool to track my tasks. I communicated with my team to delegate the report preparation, which allowed us to meet both deadlines effectively.
Can you describe a time when you took the initiative to learn a new analytical technique or tool to improve your work?
How to Answer
- 1
Identify a specific tool or technique you learned.
- 2
Explain the context of your work where this was applicable.
- 3
Describe the steps you took to learn it, such as online courses or tutorials.
- 4
Mention how you applied this knowledge to improve your results.
- 5
Share the positive outcome or impact on your work or team.
Example Answers
In my previous role as a data analyst, I noticed we were using outdated statistical techniques. I took the initiative to learn R through an online course, dedicating weekends to practice. I applied R to automate our data analysis process, which reduced our analysis time by 40% and increased accuracy.
Tell me about a time when you led a team on an analytics project. What leadership skills did you use and how did it affect the project outcome?
How to Answer
- 1
Choose a specific project and describe your role and responsibilities.
- 2
Highlight key leadership skills used, such as communication or delegation.
- 3
Explain the impact of your leadership on the team's performance and project success.
- 4
Use metrics or outcomes to quantify the success of the project.
- 5
Reflect on what you learned from the experience about leading teams.
Example Answers
In my last role, I led a team of 5 data analysts on a project to improve customer segmentation. I used clear communication to set expectations, encouraged collaboration by holding regular check-ins, and delegated tasks based on team strengths. The project led to a 15% increase in targeted marketing efficiency, as measured by the resulting campaign performance.
Describe a situation where a project requirement changed suddenly. How did you adapt and ensure successful project completion?
How to Answer
- 1
Identify the specific change in requirement clearly
- 2
Explain your initial reaction and analysis of the situation
- 3
Describe how you communicated with your team or stakeholders
- 4
Discuss the steps you took to adapt your approach or methodology
- 5
Highlight the positive outcome or lesson learned from the experience
Example Answers
In a recent project, our client changed the data model just a week before the deadline. I quickly assessed the impact on our timeline, communicated with the team about the new requirements, and we held a brainstorming session to reallocate tasks. We focused on the most critical areas that needed adjustment and managed to deliver a revised project that met the new requirements ahead of schedule.
Give an example of a time when your attention to detail prevented a major issue in an analysis.
How to Answer
- 1
Think of a specific instance in your work experience.
- 2
Describe the analysis and highlight the detail you noticed.
- 3
Explain the potential issue that was avoided.
- 4
Emphasize the impact of your attention to detail.
- 5
Keep it concise and focused on your role.
Example Answers
In my last project, while validating the data input for a regression analysis, I noticed a typo in a key variable. If it went unnoticed, it would have skewed the results and misled stakeholders. By correcting it early, we ensured the analysis was accurate and the decision-making was based on reliable data.
Don't Just Read Analytical Statistician Questions - Practice Answering Them!
Reading helps, but actual practice is what gets you hired. Our AI feedback system helps you improve your Analytical Statistician interview answers in real-time.
Personalized feedback
Unlimited practice
Used by hundreds of successful candidates
Technical Interview Questions
Explain the key differences between supervised and unsupervised learning and give examples of when you would use each.
How to Answer
- 1
Define supervised learning and unsupervised learning clearly.
- 2
Highlight the role of labeled data in supervised learning.
- 3
Mention examples of algorithms used in both methods.
- 4
Explain common use cases for each type to illustrate their applications.
- 5
Keep the answer concise, focusing on clarity and key distinctions.
Example Answers
Supervised learning involves training a model on labeled data, where the outcome is known. For example, predicting house prices uses regression techniques. Unsupervised learning, on the other hand, deals with unlabeled data to identify patterns, like using clustering to segment customers based on behavior.
What tools and techniques do you use for data visualization and why are they effective?
How to Answer
- 1
Identify key tools you are proficient in like Tableau, Power BI, or Python libraries.
- 2
Explain why the chosen tools are suitable for the type of data you're working with.
- 3
Mention specific techniques like bar graphs, scatter plots, or heat maps to highlight insights.
- 4
Connect the effectiveness of these tools to improving decision-making or storytelling.
- 5
Share an example where your visualization led to actionable insights.
Example Answers
I primarily use Tableau for data visualization because it allows for interactive dashboards that make data exploration intuitive. Techniques I prefer include bar graphs and scatter plots, which help to clearly display trends. In a recent project, I used a heat map to show customer density, which led to strategic decisions about store placements.
Don't Just Read Analytical Statistician Questions - Practice Answering Them!
Reading helps, but actual practice is what gets you hired. Our AI feedback system helps you improve your Analytical Statistician interview answers in real-time.
Personalized feedback
Unlimited practice
Used by hundreds of successful candidates
Which programming languages are you proficient in for statistical analysis and why do you prefer them?
How to Answer
- 1
Identify the languages you use most frequently for statistical tasks.
- 2
Mention specific libraries or frameworks associated with each language.
- 3
Explain why you prefer each language, focusing on its features and advantages.
- 4
Consider mentioning any relevant projects where you applied these languages.
- 5
Keep your answer concise and tailored to the job description.
Example Answers
I am proficient in R and Python for statistical analysis. I prefer R because of its extensive libraries like ggplot2 for data visualization and dplyr for data manipulation, which support efficient analysis. Python, on the other hand, is great for integrating statistical analysis into larger applications, especially with libraries like pandas and scikit-learn. In my last project, I used R to analyze survey data and Python to create machine learning models.
How do you determine the appropriate hypothesis test to use for a given dataset and research question?
How to Answer
- 1
Identify the type of data: categorical or numerical.
- 2
Determine if the data meets the assumptions for parametric tests.
- 3
Define your research question clearly: is it a comparison or a correlation?
- 4
Consider the sample size and number of groups being compared.
- 5
Choose a test that matches your data type and research design, such as t-test for two groups or ANOVA for more.
Example Answers
To determine the appropriate hypothesis test, I first assess whether my data is categorical or numerical. For example, if I'm comparing means between two groups, I would use a t-test, but if I have more than two groups, I'd opt for ANOVA. I also check assumptions like normality and equal variances.
What is multicollinearity in regression analysis, and how can it affect the results of a model?
How to Answer
- 1
Define multicollinearity clearly and briefly.
- 2
Explain its causes, such as high correlation among independent variables.
- 3
Discuss its effects on regression coefficients, such as inflated standard errors.
- 4
Mention methods to detect multicollinearity, like variance inflation factor (VIF).
- 5
Conclude with potential solutions, such as removing or combining variables.
Example Answers
Multicollinearity occurs when independent variables in a regression model are highly correlated with each other. This can lead to unreliable estimates of coefficients, making it difficult to determine the effect of each variable. Detection can be performed using the variance inflation factor, and one solution is to remove or combine correlated variables.
What are some common pitfalls in machine learning modeling, and how can they be avoided?
How to Answer
- 1
Identify the pitfalls like overfitting, underfitting, and data leakage.
- 2
Explain the importance of proper data preprocessing and feature selection.
- 3
Discuss the need for tuning hyperparameters effectively.
- 4
Mention the significance of cross-validation to assess model performance.
- 5
Highlight the value of understanding the problem space and model assumptions.
Example Answers
Common pitfalls include overfitting, where the model learns noise rather than signal. It can be avoided by using regularization techniques and cross-validation. Data leakage is another issue, often arising from improper data handling, which can be mitigated by clearly separating training and test datasets.
How do you apply the concept of Bayes' Theorem in real-world statistical analysis?
How to Answer
- 1
Explain Bayes' Theorem simply and its relevance.
- 2
Provide a specific example of its application in a field, such as healthcare or finance.
- 3
Mention how Bayes' Theorem helps in updating probabilities with new evidence.
- 4
Highlight the importance of prior distributions in your example.
- 5
Keep your explanation focused and avoid overly technical jargon.
Example Answers
In healthcare, I apply Bayes' Theorem to update the probability of a patient having a disease as new test results come in, using prior knowledge of the disease's prevalence.
What are the main challenges when dealing with big data and how do you overcome them?
How to Answer
- 1
Identify common challenges like data quality, processing speed, and storage capacity.
- 2
Discuss the importance of using the right tools and technologies for data analysis.
- 3
Emphasize the need for effective data cleaning and preprocessing strategies.
- 4
Mention collaboration with cross-functional teams for better insights.
- 5
Highlight your experience with scalable architectures and data management solutions.
Example Answers
One main challenge is data quality. I ensure high quality by employing robust data cleaning techniques and validating data sources before analysis.
How do you design an experiment to test a hypothesis, and what factors do you consider?
How to Answer
- 1
Define your hypothesis clearly and ensure it's testable.
- 2
Identify the independent and dependent variables in your experiment.
- 3
Determine the sample size needed for reliable results.
- 4
Consider control groups to account for external factors.
- 5
Plan the methods for data collection and analysis upfront.
Example Answers
I start by clearly defining the hypothesis I want to test, ensuring it is specific and measurable. I then identify the independent variable I'll manipulate and the dependent variable I'll measure. For example, if I'm testing a drug's effectiveness, the drug dose is independent and the health outcome is dependent. I calculate the needed sample size based on power analysis to ensure my results will be significant. A control group is essential to compare against the treatment group, allowing me to isolate the effect of the independent variable. Finally, I outline the data collection methods, whether through surveys, experiments, or observational studies.
What are some ethical considerations one should keep in mind while conducting statistical analysis?
How to Answer
- 1
Ensure data confidentiality and privacy for all subjects
- 2
Avoid bias in data collection and interpretation
- 3
Be transparent about methodologies and limitations of analysis
- 4
Provide informed consent when involving human subjects
- 5
Report findings honestly without manipulating data to fit a narrative
Example Answers
One key ethical consideration is ensuring data confidentiality; for instance, anonymizing data sources helps protect individual privacy. Additionally, avoiding bias is crucial; if I'm using surveys, I would ensure the questions are neutral and represent diverse perspectives.
Don't Just Read Analytical Statistician Questions - Practice Answering Them!
Reading helps, but actual practice is what gets you hired. Our AI feedback system helps you improve your Analytical Statistician interview answers in real-time.
Personalized feedback
Unlimited practice
Used by hundreds of successful candidates
Situational Interview Questions
You receive a dataset with missing values and outliers. How would you handle this situation to ensure the reliability of your analysis?
How to Answer
- 1
Identify the extent and pattern of missing values in the dataset.
- 2
Decide on an imputation method for missing values, such as mean, median, or model-based.
- 3
Use visualizations like boxplots to detect and assess outliers.
- 4
Determine whether to remove outliers or adjust them based on their impact on your analysis.
- 5
Document your decisions and the rationale behind your methods for transparency.
Example Answers
First, I would analyze the missing values to see if they are random or systematic. Then, I might use mean imputation for numerical data, or mode for categorical data. For outliers, I would use boxplots to visualize their impact and decide whether to remove them or cap them to prevent distortion of results.
Imagine you have three different models that give three different results for the same dataset. How would you determine which model to use?
How to Answer
- 1
Compare model performance metrics like accuracy, precision, recall, and F1 score.
- 2
Consider the context of the problem and the implications of the results each model produces.
- 3
Verify model assumptions and ensure they are satisfied by the dataset.
- 4
Perform cross-validation to check the stability of each model's performance.
- 5
Assess the interpretability and complexity of each model based on the use case.
Example Answers
I would first evaluate the performance metrics of each model, such as accuracy and F1 score, to see which one performs better. Then I would check if the model assumptions are met by the data to ensure valid results.
Don't Just Read Analytical Statistician Questions - Practice Answering Them!
Reading helps, but actual practice is what gets you hired. Our AI feedback system helps you improve your Analytical Statistician interview answers in real-time.
Personalized feedback
Unlimited practice
Used by hundreds of successful candidates
You've been asked to manipulate data to support a biased narrative. How would you handle this ethical dilemma?
How to Answer
- 1
Identify the ethical implications of manipulating data
- 2
Express the importance of data integrity and accuracy
- 3
Suggest alternative ways to present data without bias
- 4
Offer to discuss the objective conclusions based on the data
- 5
Propose consulting with a supervisor or ethics board if necessary
Example Answers
I would explain to my supervisor that manipulating data compromises integrity and can have serious consequences. I'd suggest presenting the data as it is and letting the facts speak for themselves.
You are tasked with developing a new statistical model for predicting customer churn. How would you go about this process?
How to Answer
- 1
Define the problem and clarify objectives for the model.
- 2
Collect and explore customer data including demographics, behavior, and previous churn instances.
- 3
Choose appropriate statistical methods, such as logistic regression or decision trees.
- 4
Evaluate model performance using metrics like accuracy, precision, and recall.
- 5
Iterate on the model by refining based on feedback and new data.
Example Answers
First, I would clarify the goal of the model, focusing on what factors specifically contribute to churn. Next, I would gather relevant customer data, look for patterns, and preprocess it for analysis. I would consider methods like logistic regression for prediction. After building the model, I would assess its performance with metrics such as accuracy and refine it based on results.
A client is disappointed with the results of your analysis. How do you handle the situation and address their concerns?
How to Answer
- 1
Listen actively to the client's concerns without interrupting.
- 2
Acknowledge their feelings and validate their disappointment.
- 3
Clarify the analysis results and explain any limitations transparently.
- 4
Propose next steps to address their issues or provide additional insights.
- 5
Follow up with them after the discussion to ensure satisfaction.
Example Answers
I start by listening carefully to the client's concerns and making sure they feel heard. I acknowledge their disappointment and explain the results in clear terms, including any limitations. Then, I suggest next steps, such as a follow-up analysis or additional data exploration, and I ensure to check in with them afterward to see how they feel.
You have limited computational resources for a big data project. How would you prioritize and manage the analysis?
How to Answer
- 1
Identify key objectives and metrics for the analysis.
- 2
Select a subset of the data that addresses your main questions.
- 3
Use sampling techniques to reduce the data size efficiently.
- 4
Prioritize algorithms that are computationally efficient.
- 5
Run exploratory data analysis to inform more focused analyses later.
Example Answers
I would start by defining the key objectives of the project and the specific metrics I need to analyze. Then, I'd select a relevant subset of the data that captures these objectives. Using sampling methods, I would reduce the data size while maintaining representativeness and focus on computationally efficient algorithms to get quick insights.
You are asked to perform a risk assessment on a new product launch. How would you approach this analysis?
How to Answer
- 1
Identify potential risks associated with the product launch including market, operational, and financial risks
- 2
Use quantitative methods to assess the likelihood and impact of each risk
- 3
Engage with stakeholders to gather insights and validate risk assumptions
- 4
Develop mitigation strategies for high-priority risks
- 5
Document your findings in a clear report for decision-makers
Example Answers
I would start by brainstorming possible risks with my team, considering factors like market competition and operational challenges. Then, I would quantify these risks using statistical models to measure their potential impacts. I would also consult key stakeholders to ensure we have a comprehensive view of the risks. Following this, I would outline strategies to mitigate the most significant risks and summarize my analysis in a report for the management team.
You're collaborating with a team that lacks statistical expertise. How do you ensure effective analysis and communication?
How to Answer
- 1
Assess the team's existing knowledge and tailor your explanations accordingly
- 2
Use visualizations and clear graphs to present data insights effectively
- 3
Break down complex statistical concepts into simpler, relatable terms
- 4
Encourage questions and foster an open dialogue for better understanding
- 5
Set regular check-ins to ensure alignment and clarity throughout the process
Example Answers
I would start by understanding the team's background and knowledge level, then present analysis using simple visualizations. I’d make sure to explain concepts in plain terms and regularly check in to address any questions they have.
You need to provide real-time analytics for live data streams. What challenges do you anticipate and how would you address them?
How to Answer
- 1
Identify key challenges such as data volume, latency, and data quality issues.
- 2
Discuss strategies for managing data throughput and ensuring timely processing.
- 3
Highlight tools or technologies you would use for real-time analytics.
- 4
Emphasize the importance of monitoring and continuous feedback loops.
- 5
Mention collaboration with cross-functional teams for data insights.
Example Answers
In handling real-time analytics, I anticipate challenges like high data volume and latency. To address these, I would implement stream processing frameworks like Apache Kafka or Spark Streaming to manage data efficiently. Monitoring tools will help keep track of performance and I would establish feedback loops to continually improve our models.
During a presentation, key stakeholders challenge the validity of your analysis. How do you respond and maintain confidence?
How to Answer
- 1
Stay calm and listen actively to their concerns.
- 2
Acknowledge their perspective and thank them for their input.
- 3
Present evidence from your analysis to support your findings.
- 4
Ask clarifying questions to understand their objections better.
- 5
Maintain professionalism and confidence in your expertise.
Example Answers
I would begin by calmly listening to their concerns and acknowledging their input. Then, I would present the evidence from my analysis, explaining the methodology and data sources clearly to support my findings.
Don't Just Read Analytical Statistician Questions - Practice Answering Them!
Reading helps, but actual practice is what gets you hired. Our AI feedback system helps you improve your Analytical Statistician interview answers in real-time.
Personalized feedback
Unlimited practice
Used by hundreds of successful candidates
Analytical Statistician Position Details
Related Positions
Ace Your Next Interview!
Practice with AI feedback & get hired faster
Personalized feedback
Used by hundreds of successful candidates
Ace Your Next Interview!
Practice with AI feedback & get hired faster
Personalized feedback
Used by hundreds of successful candidates