Top 29 Statistical Data Analyst Interview Questions and Answers [Updated 2025]
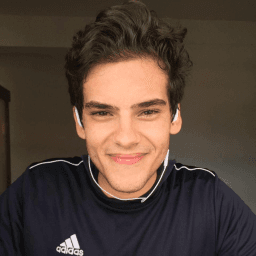
Andre Mendes
•
March 30, 2025
Preparing for a Statistical Data Analyst interview? Our updated guide offers a comprehensive collection of the most common questions you might face, complete with example answers and expert tips to help you respond effectively. Whether you’re a seasoned professional or a newcomer, this post is designed to boost your confidence and ensure you're ready to impress your interviewers with your analytical prowess.
Download Statistical Data Analyst Interview Questions in PDF
To make your preparation even more convenient, we've compiled all these top Statistical Data Analystinterview questions and answers into a handy PDF.
Click the button below to download the PDF and have easy access to these essential questions anytime, anywhere:
List of Statistical Data Analyst Interview Questions
Behavioral Interview Questions
Tell me about a time when you had to work closely with a team to complete a statistical analysis project. What was your role, and how did you contribute to the team's success?
How to Answer
- 1
Choose a specific project where teamwork was essential
- 2
Clearly define your role and responsibilities within the team
- 3
Mention the tools or methodologies you used for the analysis
- 4
Highlight a challenge the team faced and how you contributed to overcoming it
- 5
Explain the outcome of the project and its impact or success
Example Answers
In my previous role, we worked on a project analyzing customer behavior data to improve marketing strategies. I was responsible for data cleaning and performing exploratory data analysis using Python. A challenge we faced was integrating data from different sources, but I suggested using consistent data formats, which streamlined our work. The final analysis led to a 15% increase in campaign effectiveness.
Describe a challenging analytical problem you encountered in your past work. How did you approach solving it?
How to Answer
- 1
Identify the specific analytical problem you faced
- 2
Explain the data you used and tools or methods applied
- 3
Discuss the steps you took to analyze the problem
- 4
Highlight any challenges you encountered in the process
- 5
Mention the outcome or what you learned from it
Example Answers
In my previous role, we faced a problem with declining customer engagement metrics. I gathered data from our analytics platform, used Python to perform a cohort analysis, and identified that a specific segment was disengaging. I proposed a targeted marketing campaign that increased engagement by 25% over the next quarter.
Don't Just Read Statistical Data Analyst Questions - Practice Answering Them!
Reading helps, but actual practice is what gets you hired. Our AI feedback system helps you improve your Statistical Data Analyst interview answers in real-time.
Personalized feedback
Unlimited practice
Used by hundreds of successful candidates
Have you ever led a project where you had to analyze complex data sets? How did you manage the project and ensure successful completion?
How to Answer
- 1
Describe a specific project you led
- 2
Explain your role and responsibilities clearly
- 3
Highlight the tools and methods you used for data analysis
- 4
Discuss how you coordinated with team members and stakeholders
- 5
Mention the outcome and what you learned from the experience
Example Answers
In my previous role, I led a project analyzing customer feedback data. I coordinated a team of three analysts and used SQL and Python for data cleaning and analysis. I scheduled weekly check-ins to ensure we stayed on track and adjusted our approach based on insights. The project resulted in a 15% increase in customer satisfaction after we implemented changes based on our findings.
Can you provide an example of a time when you had to explain statistical findings to a non-technical audience? How did you ensure they understood the results?
How to Answer
- 1
Identify a specific situation where you explained findings.
- 2
Use simple language and avoid jargon.
- 3
Incorporate visuals or analogies to clarify complex concepts.
- 4
Check for understanding by inviting questions.
- 5
Summarize key findings in a clear and concise manner.
Example Answers
In my previous role, I presented survey results to the marketing team. I used bar charts to show customer preferences and explained the significance of each finding in layman's terms. After the presentation, I encouraged team members to ask questions, which helped clarify their understanding.
Tell me about a time when you had to quickly learn a new statistical tool or software. How did you handle the situation?
How to Answer
- 1
Identify a specific tool you learned quickly
- 2
Explain the context or challenge that required learning it
- 3
Describe the steps you took to learn the tool
- 4
Highlight the outcome or what you achieved
- 5
Reflect on any skills that helped you learn fast
Example Answers
In my previous job, we had a sudden requirement to use R for data analysis instead of Python. I enrolled in an online course and followed tutorials for a week, practicing daily. Within two weeks, I completed a project that improved our reporting process and received positive feedback from my manager.
Give me an example of a project where attention to detail was crucial in achieving accurate results.
How to Answer
- 1
Choose a specific project related to data analysis.
- 2
Highlight the importance of data accuracy in your example.
- 3
Explain how attention to detail influenced the project outcomes.
- 4
Mention any tools or methods you used to ensure detail-oriented work.
- 5
Conclude with the impact of your attention to detail on stakeholders or results.
Example Answers
In my previous role, I worked on a customer segmentation project. I meticulously cleaned the data, ensuring no duplicates or missing values. This attention to detail allowed us to accurately identify target demographics, which improved our marketing strategy by 20%.
Describe a time when you developed a new approach to solve a complex data analysis problem.
How to Answer
- 1
Identify a specific problem you faced in data analysis.
- 2
Explain the limitations of traditional methods you initially used.
- 3
Describe the new approach you developed logically.
- 4
Highlight the results achieved from your new approach.
- 5
Reflect on what you learned from the experience.
Example Answers
In my previous role, I was tasked with analyzing customer churn data, but standard regression models weren't capturing key variables. I developed a machine learning model using decision trees, which allowed me to include interaction effects. This new approach increased our prediction accuracy by 25%, helping the company reduce churn by implementing targeted interventions. I learned the importance of adapting our methods to the data's complexity.
How have you balanced multiple projects or tasks that required extensive data analysis?
How to Answer
- 1
Prioritize tasks based on deadlines and impact
- 2
Use project management tools to track progress
- 3
Break the analysis into smaller, manageable parts
- 4
Communicate with stakeholders to align expectations
- 5
Regularly review and adjust your workload as needed
Example Answers
In my previous role, I prioritized projects by impact and urgency, which helped me focus on the most critical analyses first. I used Trello to track my progress and break down tasks into smaller parts, which made them easier to manage.
Can you give an example of receiving feedback on your analysis work? How did you incorporate it into your process?
How to Answer
- 1
Choose a specific project where you received feedback.
- 2
Describe the nature of the feedback clearly.
- 3
Explain how you adjusted your analysis based on the feedback.
- 4
Highlight any positive outcomes that resulted from incorporating the feedback.
- 5
Mention how this experience improved your overall skills in analysis.
Example Answers
In a recent project analyzing customer satisfaction data, I received feedback from my supervisor that my initial results lacked clarity. I revised my visualizations to better highlight trends and key insights, which led to a more impactful presentation for stakeholders.
Describe a situation where you had to make a critical decision based on incomplete or conflicting data.
How to Answer
- 1
Identify a specific situation from your experience.
- 2
Explain the context and what data was conflicting or incomplete.
- 3
Describe the decision-making process you used.
- 4
Discuss the outcome of your decision and what you learned.
- 5
Keep it concise and focused on your role.
Example Answers
In my previous job, I was tasked with analyzing customer feedback data that contained both positive and negative comments. There was conflicting data regarding customer satisfaction. I decided to prioritize the quantitative scores of the surveys over anecdotal comments to guide product changes, which resulted in a 15% increase in satisfaction within three months.
Don't Just Read Statistical Data Analyst Questions - Practice Answering Them!
Reading helps, but actual practice is what gets you hired. Our AI feedback system helps you improve your Statistical Data Analyst interview answers in real-time.
Personalized feedback
Unlimited practice
Used by hundreds of successful candidates
Technical Interview Questions
What statistical techniques do you find most effective for analyzing large datasets, and why?
How to Answer
- 1
Identify statistical techniques relevant to large datasets such as regression analysis, clustering, or hypothesis testing.
- 2
Explain the reason for choosing each technique based on dataset characteristics.
- 3
Use specific examples from your experience to illustrate effectiveness.
- 4
Mention tools or software you use to apply these techniques.
- 5
Highlight any insights or outcomes achieved using these methods.
Example Answers
I find regression analysis particularly effective for large datasets as it helps in understanding relationships between variables. For instance, while analyzing sales data, I used linear regression to find factors affecting sales and increased our marketing ROI by 20%.
How do you approach the process of data cleaning and preparation before conducting analysis?
How to Answer
- 1
Identify missing values and decide how to handle them
- 2
Remove duplicate entries to ensure data integrity
- 3
Standardize formats for consistency, such as dates and categories
- 4
Apply outlier detection methods to ensure accuracy of analysis
- 5
Document all cleaning steps for reproducibility and transparency
Example Answers
I start by examining the dataset for missing values and decide whether to fill them or remove the affected rows. I also check for duplicate records to ensure the dataset is unique, standardize date formats, and look for any outliers that might skew results. Finally, I take note of all changes I make for future reference.
Don't Just Read Statistical Data Analyst Questions - Practice Answering Them!
Reading helps, but actual practice is what gets you hired. Our AI feedback system helps you improve your Statistical Data Analyst interview answers in real-time.
Personalized feedback
Unlimited practice
Used by hundreds of successful candidates
What statistical software and tools are you proficient with, and how have you used them in past projects?
How to Answer
- 1
Identify key software relevant to statistical analysis like R, Python, SAS, or SPSS.
- 2
Mention specific projects where you applied these tools to solve real problems.
- 3
Highlight any advanced features or techniques you used within the software.
- 4
Discuss collaboration with team members using these tools for effective outcomes.
- 5
Be ready to explain the impact of your work using these tools in measurable ways.
Example Answers
I am proficient with R and Python. In my last project, I used R to analyze large datasets for trend forecasting, utilizing packages like dplyr and ggplot2 for data manipulation and visualization.
Can you explain how you would build a predictive model using linear regression?
How to Answer
- 1
Define the problem and the target variable clearly.
- 2
Collect and preprocess the data, handling missing values and outliers.
- 3
Choose relevant features and perform exploratory data analysis to understand relationships.
- 4
Train the linear regression model using a suitable library or software.
- 5
Evaluate the model's performance using metrics like R-squared and RMSE, and make adjustments if necessary.
Example Answers
First, I would identify the target variable I want to predict and ensure I understand the problem. Then, I would gather and preprocess my dataset, making sure to clean it by removing any missing values and addressing outliers. I'd perform exploratory data analysis to select relevant features that correlate well with the target. After that, I'd use a library like scikit-learn to train a linear regression model. Finally, I'd evaluate the model's performance with R-squared and RMSE to confirm accuracy.
How do you determine which statistical test is appropriate to validate a hypothesis?
How to Answer
- 1
Identify the type of data: categorical or continuous.
- 2
Determine the number of groups being compared.
- 3
Assess the distribution of the data: normal or non-normal.
- 4
Consider whether the samples are independent or related.
- 5
Know the assumptions of each statistical test before choosing.
Example Answers
To determine the appropriate statistical test, I first identify the type of data I have. If it's categorical, I might use a Chi-square test. For continuous data, I would consider a t-test if comparing two groups or ANOVA for more than two groups, checking for normal distribution first.
Can you describe a complex data manipulation task you performed using R? How did you use the language to accomplish this?
How to Answer
- 1
Identify a specific project or task that involved data manipulation.
- 2
Explain the dataset and the complexity involved.
- 3
Describe the R functions or libraries you used.
- 4
Highlight the steps you took to transform the data.
- 5
Mention the outcome or result of your manipulation.
Example Answers
I worked on a project where I had to clean and analyze a large dataset of customer transactions. Using the 'dplyr' library, I filtered the data to only include purchases over $100, grouped by customer ID, and calculated the total spending for each customer. This helped identify our top customers.
How would you approach building a data pipeline in Python for a statistical analysis task?
How to Answer
- 1
Identify the data sources and formats you will use.
- 2
Use libraries like pandas for data manipulation and cleaning.
- 3
Implement a way to automate data extraction, transformation, and loading (ETL).
- 4
Utilize tools like Airflow for scheduling and managing the pipeline.
- 5
Consider how to validate data quality at each stage.
Example Answers
First, I would identify the data sources, such as CSV files or API endpoints. Then, I'd use pandas to clean and manipulate the data. I would set up an ETL process to automate data retrieval and loading into a database. To manage the pipeline, I would use Airflow for scheduling, and I'd implement checks to ensure data quality throughout.
What are some challenges of analyzing big data sets and how have you addressed them in your past work?
How to Answer
- 1
Identify common challenges such as data quality, scale, and processing speed.
- 2
Use specific examples from your experience to illustrate how you tackled these challenges.
- 3
Mention tools or techniques you used, like data cleaning methods or frameworks.
- 4
Emphasize collaboration with cross-functional teams to solve complex issues.
- 5
Highlight the impact of your solutions on the project outcomes.
Example Answers
In my previous role, I faced challenges with the sheer volume of data, which sometimes led to slow processing times. To address this, I implemented parallel processing using Apache Spark, which reduced our analysis time by 40%.
What tools do you use for data visualization, and how do you decide which visualization best represents your data?
How to Answer
- 1
Identify key tools you are proficient in, such as Tableau, Power BI, or Python libraries like Matplotlib and Seaborn.
- 2
Discuss how you match visualizations to the type of data and the story you want to convey.
- 3
Mention the importance of your audience and their preferences in choosing a visualization type.
- 4
Include any experience with interactive visualizations or dashboards if applicable.
- 5
Share an example of a project where a specific visualization made a significant impact.
Example Answers
I primarily use Tableau for interactive dashboards and Matplotlib for static visualizations. I choose visualizations based on the data distribution and insights I want to highlight. For instance, I used a combination of bar charts and scatter plots to showcase sales trends and customer segmentation in a recent project.
Have you used machine learning algorithms in your work? Can you provide an example of how they improved your analysis?
How to Answer
- 1
Identify a specific machine learning algorithm you used.
- 2
Describe the context of the analysis and the data involved.
- 3
Explain how the algorithm was implemented and its role.
- 4
Highlight the impact or improvement resulting from the algorithm.
- 5
Keep the example clear and focused on your contribution.
Example Answers
In my previous role, I used a random forest algorithm to analyze customer churn. We had a dataset with customer demographics and usage patterns. By implementing this model, we identified key factors leading to churn, which helped the marketing team target high-risk customers effectively, resulting in a 10% reduction in churn rate.
Don't Just Read Statistical Data Analyst Questions - Practice Answering Them!
Reading helps, but actual practice is what gets you hired. Our AI feedback system helps you improve your Statistical Data Analyst interview answers in real-time.
Personalized feedback
Unlimited practice
Used by hundreds of successful candidates
Situational Interview Questions
Imagine you discover an anomaly in the data that could potentially affect the analysis outcomes. What steps would you take to address this issue?
How to Answer
- 1
Confirm the anomaly by re-checking the data for accuracy.
- 2
Investigate potential causes of the anomaly, such as data entry errors or outlier influences.
- 3
Document the anomaly and your findings clearly.
- 4
Consult with relevant stakeholders or team members for additional insights.
- 5
Decide on a corrective action or communicate the implications of the anomaly on the analysis.
Example Answers
First, I would double-check the data to confirm the anomaly is not due to a mistake in processing. Then, I'd analyze possible reasons, like data entry issues or unexpected outliers. I would document my findings and discuss them with my team to decide if we need to adjust the dataset or communicate the potential impact on the final analysis.
You are given a tight deadline to complete a statistical analysis project. How would you prioritize tasks to ensure timely delivery?
How to Answer
- 1
Identify critical tasks that must be completed first.
- 2
Break down the project into smaller, manageable parts.
- 3
Assess the time each task will take and allocate resources accordingly.
- 4
Use project management tools to track progress and deadlines.
- 5
Communicate with stakeholders about priorities and any potential delays.
Example Answers
I would start by identifying the key deliverables of the project and focus on tasks that are essential for completion. After breaking down the analysis into smaller parts, I would estimate the time for each and prioritize based on their impact on the final deliverable.
Don't Just Read Statistical Data Analyst Questions - Practice Answering Them!
Reading helps, but actual practice is what gets you hired. Our AI feedback system helps you improve your Statistical Data Analyst interview answers in real-time.
Personalized feedback
Unlimited practice
Used by hundreds of successful candidates
Suppose you and a colleague have differing opinions on the analysis method to use for a project. How would you handle the situation?
How to Answer
- 1
Listen actively to your colleague's reasoning and viewpoints
- 2
Present your perspective clearly and with data support
- 3
Look for common ground or shared goals in the project
- 4
Suggest a trial of both methods to compare results
- 5
Stay professional and focus on finding the best solution for the project
Example Answers
I would first listen to my colleague's approach to understand their reasoning. Then, I would present my method and back it up with relevant data. If we still disagree, I might suggest we test both methods and see which yields better results.
If your analysis results contradict the expectations of a senior stakeholder, how would you validate and communicate your findings?
How to Answer
- 1
Review the analysis methodology and ensure accuracy through re-checking calculations and data sources
- 2
Use visual aids like graphs to present data clearly and emphasize unexpected findings
- 3
Prepare to explain the context and rationale behind the results to align with the stakeholder's expectations
- 4
Anticipate questions or concerns and prepare clear responses supported by data
- 5
Engage the stakeholder in a discussion to understand their perspective and address their concerns effectively
Example Answers
I would carefully review my analysis to ensure there are no errors, then create a visual presentation to clearly show my findings. I would explain the analysis process and provide context to the stakeholder.
How would you handle a situation where a client requests an analysis based on incomplete or flawed data?
How to Answer
- 1
Assess the quality and completeness of the data before proceeding.
- 2
Communicate transparently with the client about data issues.
- 3
Suggest alternative approaches or adjustments based on the data available.
- 4
Provide insights on the potential impact of using flawed data.
- 5
Document any limitations and assumptions in your analysis report.
Example Answers
First, I would evaluate the data for any gaps or inconsistencies. Then, I would inform the client of these issues and discuss how we might work with the data to extract useful insights. I could suggest using a different method, like a qualitative approach, if the data is too flawed for a quantitative analysis.
If you identified inefficiencies in the data analysis process, what steps would you take to optimize it?
How to Answer
- 1
Conduct a thorough analysis of the existing workflow to identify bottlenecks
- 2
Consult with team members to gather insights on pain points
- 3
Implement automation tools for repetitive tasks
- 4
Establish clear documentation for data processes and protocols
- 5
Regularly review and update the process based on feedback and results
Example Answers
I would first analyze the current workflow to see where delays occur and then talk to team members for additional insights. If tasks are repetitive, I'd explore automation solutions to save time. Finally, I'd keep the documentation up to date to ensure everyone is on the same page.
How would you approach a project where you need to collaborate with engineers and marketing to analyze product data?
How to Answer
- 1
Identify the objectives of the analysis with marketing and engineering teams.
- 2
Schedule regular meetings to ensure alignment and communication.
- 3
Use a common data platform to share insights and findings.
- 4
Define key metrics that are important to both engineering and marketing.
- 5
Be open to feedback and adapt your analysis based on team needs.
Example Answers
I would start by discussing the goals of the analysis with both the marketing and engineering teams. Then, I would set up bi-weekly check-in meetings to track our progress and maintain clear communication. Using a shared data visualization tool would help us access real-time insights together. It's important that we agree on metrics that reflect both marketing effectiveness and engineering performance, ensuring we all have a unified focus.
What would you do if you were asked to manipulate data to achieve a desired outcome in your analysis?
How to Answer
- 1
Acknowledge the importance of ethical standards in data analysis.
- 2
Emphasize the significance of integrity in reporting results.
- 3
Discuss the importance of using appropriate methods to analyze data.
- 4
Mention the need for transparency in the analysis process.
- 5
Propose to consult with stakeholders to understand the analysis goals.
Example Answers
If asked to manipulate data, I would first clarify the analysis goals with stakeholders, ensuring I understand their needs. I would then apply statistical methods that maintain integrity and transparency. Maintaining ethical standards is crucial, so I would avoid any manipulation that misrepresents the data.
Given limited resources, how would you ensure high-quality analysis on a critical project?
How to Answer
- 1
Prioritize key analytical tasks that have the most impact.
- 2
Leverage existing data and tools to reduce workload.
- 3
Use a structured methodology to maintain focus and efficiency.
- 4
Collaborate with team members for shared insights and expertise.
- 5
Continuously validate and check results to ensure accuracy.
Example Answers
I would first identify the most crucial analyses that drive project goals and focus our limited resources on those. Then, I would use existing databases and analytical tools to streamline the process, ensuring we don't reinvent the wheel.
Statistical Data Analyst Position Details
Recommended Job Boards
CareerBuilder
www.careerbuilder.com/jobs-statistical-data-analystThese job boards are ranked by relevance for this position.
Related Positions
Ace Your Next Interview!
Practice with AI feedback & get hired faster
Personalized feedback
Used by hundreds of successful candidates
Ace Your Next Interview!
Practice with AI feedback & get hired faster
Personalized feedback
Used by hundreds of successful candidates