Top 30 Mathematical Statistician Interview Questions and Answers [Updated 2025]
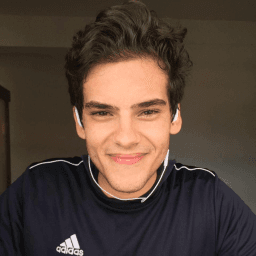
Andre Mendes
•
March 30, 2025
Preparing for a Mathematical Statistician interview? This comprehensive guide covers the most common questions aspiring statisticians face, complete with example answers and expert tips to help you respond with confidence. Whether you're a seasoned professional or a recent graduate, these insights will equip you to showcase your analytical skills and statistical knowledge effectively. Dive in and get ready to ace your interview!
Download Mathematical Statistician Interview Questions in PDF
To make your preparation even more convenient, we've compiled all these top Mathematical Statisticianinterview questions and answers into a handy PDF.
Click the button below to download the PDF and have easy access to these essential questions anytime, anywhere:
List of Mathematical Statistician Interview Questions
Behavioral Interview Questions
Describe a time when you had to work closely with a team to complete a statistical analysis project. What was the project and how did you contribute to the team's success?
How to Answer
- 1
Identify a specific project that involved teamwork.
- 2
Highlight your role and specific contributions to the project.
- 3
Mention tools or methodologies used to gather and analyze data.
- 4
Emphasize the importance of collaboration and communication in your team.
- 5
Discuss the outcome and what you learned from the experience.
Example Answers
In my last role, our team worked on a project assessing customer satisfaction through survey data. I was responsible for designing the survey and conducting the statistical analysis using R. I coordinated our efforts by scheduling regular check-ins, which helped us address challenges quickly. The project resulted in actionable insights that improved our products and increased satisfaction scores by 15%.
Can you give an example of a particularly challenging statistical problem you faced in your previous work and how you approached solving it?
How to Answer
- 1
Identify a specific challenging problem you encountered.
- 2
Explain the context and why it was challenging.
- 3
Describe the methods or tools you used to tackle the problem.
- 4
Discuss the outcome and what you learned from the experience.
- 5
Be concise and focus on your critical thinking and problem-solving skills.
Example Answers
In my previous role, I faced a problem with missing data in a large survey dataset. This was challenging because it could bias our results. I first conducted a missing data analysis to understand the patterns, then used multiple imputation techniques to fill in the gaps. As a result, our model's performance improved significantly, and we were able to publish our findings with confidence.
Don't Just Read Mathematical Statistician Questions - Practice Answering Them!
Reading helps, but actual practice is what gets you hired. Our AI feedback system helps you improve your Mathematical Statistician interview answers in real-time.
Personalized feedback
Unlimited practice
Used by hundreds of successful candidates
Tell me about a time when you had to learn a new statistical method or technology quickly to apply it to your work. How did you go about it?
How to Answer
- 1
Identify a specific statistical method or technology you learned.
- 2
Describe the context of your work and the urgency of learning.
- 3
Explain your specific steps to learn quickly, like online courses or tutorials.
- 4
Mention any resources or people who assisted you in the process.
- 5
Conclude with how you successfully applied the method to your work.
Example Answers
In my previous role, I needed to learn Bayesian analysis quickly for a project. I enrolled in an online course over a weekend and utilized resources like 'Bayesian Data Analysis' books. Additionally, I reached out to a colleague who had experience in that area. Within a week, I applied Bayesian methods to improve our model's accuracy, resulting in a 10% increase in predictive performance.
Give an example of how you explained a complex statistical concept to a non-expert audience. What approach did you use?
How to Answer
- 1
Identify a specific statistical concept that is complex.
- 2
Choose a non-technical analogy to simplify the concept.
- 3
Break down the concept into basic components.
- 4
Use visual aids or examples to enhance understanding.
- 5
Encourage questions to ensure engagement and clarity.
Example Answers
I explained the concept of p-values to a group of community members by comparing it to a game of chance. I used a dice analogy to show how often you'd roll a certain number and what that means in terms of statistical significance.
Describe a situation where you used innovative statistical techniques to achieve better insights. What was the outcome?
How to Answer
- 1
Choose a specific project where you applied a novel technique.
- 2
Explain the technique clearly and why it was innovative.
- 3
Describe how the technique led to improved insights or results.
- 4
Quantify the outcomes when possible, like improved accuracy or cost savings.
- 5
Reflect on what you learned from the experience.
Example Answers
In a market research project, I applied a novel Bayesian hierarchical model to evaluate customer preferences. By incorporating prior information, we improved predictive accuracy by 15%, leading to a more targeted marketing strategy that increased sales by 10%.
Describe an experience where you led a project involving statistical analysis. What challenges did you face and how did you overcome them?
How to Answer
- 1
Choose a specific project that demonstrates your leadership in statistical analysis.
- 2
Outline the goals of the project and your role in it.
- 3
Mention specific challenges you encountered during the project.
- 4
Explain the strategies or methods you used to overcome these challenges.
- 5
Conclude with the outcomes or results of the project.
Example Answers
In my previous role, I led a project analyzing survey data for consumer preferences. The main challenge was dealing with incomplete data entries. I implemented data imputation techniques to fill in gaps and ensured the results were accurate. Ultimately, our findings helped the marketing team tailor their strategies effectively.
How do you keep your statistical skills and knowledge up to date in this rapidly evolving field?
How to Answer
- 1
Follow reputable statistical journals and publications regularly.
- 2
Participate in online courses or webinars to learn new techniques.
- 3
Join professional organizations to network and gain insights.
- 4
Engage in hands-on projects or collaborations to apply new knowledge.
- 5
Attend conferences to learn about emerging trends and tools.
Example Answers
I subscribe to journals like the Journal of the American Statistical Association, and I often take online courses on platforms like Coursera to learn the latest methodologies.
Have you ever had a disagreement with a colleague over statistical methods or analysis results? How did you resolve it?
How to Answer
- 1
Describe the situation briefly and focus on the disagreement.
- 2
Explain the statistical methods or results that were in question.
- 3
Share how you approached the conversation with your colleague.
- 4
Highlight any collaborative actions taken to resolve the disagreement.
- 5
Conclude with the result of the discussion and what you learned from it.
Example Answers
During a project, my colleague and I disagreed on the choice of hypothesis testing method. I explained my reasoning clearly regarding using a t-test instead of a non-parametric test based on the data distribution. We decided to conduct the t-test and agreed to validate the results with a non-parametric test as well. This compromise helped us both feel satisfied with the results and improved our collaboration.
Technical Interview Questions
What are the steps you follow to perform hypothesis testing? Can you explain with an example?
How to Answer
- 1
Define null and alternative hypotheses clearly.
- 2
Choose an appropriate significance level, commonly 0.05.
- 3
Select the right test based on data type and distribution.
- 4
Calculate the test statistic and p-value from data.
- 5
Draw a conclusion based on p-value and significance level.
Example Answers
To perform hypothesis testing, I first define the null hypothesis, H0, and the alternative hypothesis, H1. For instance, if I'm testing whether a new drug is effective, H0 might state that there is no effect, while H1 states that there is an effect. I would set a significance level, like 0.05, choose a t-test if the data is normally distributed, calculate the t-statistic and p-value, and if the p-value is less than 0.05, I would reject H0.
Which statistical software packages are you most familiar with, and can you discuss their strengths and weaknesses?
How to Answer
- 1
List your most familiar software packages directly
- 2
Briefly mention specific strengths and weaknesses of each
- 3
Use examples from past experience to illustrate points
- 4
Be honest about limitations while showcasing your skills
- 5
Align your software knowledge with the job requirements
Example Answers
I am most familiar with R and Python. R is great for statistical analysis and visualization with packages like ggplot2, but it can be less efficient for very large datasets. Python, on the other hand, has excellent libraries like pandas and NumPy for data manipulation, but its statistical functionality might not be as robust as R's.
Don't Just Read Mathematical Statistician Questions - Practice Answering Them!
Reading helps, but actual practice is what gets you hired. Our AI feedback system helps you improve your Mathematical Statistician interview answers in real-time.
Personalized feedback
Unlimited practice
Used by hundreds of successful candidates
Can you explain the differences between linear and logistic regression, and provide an example use case for each?
How to Answer
- 1
Define linear regression succinctly, focusing on its use with continuous outcomes.
- 2
Define logistic regression, highlighting its use for binary outcomes.
- 3
Discuss key differences in the outcome variable types and the models' outputs.
- 4
Provide a real-world example for both linear and logistic regression.
- 5
Keep explanations clear and avoid technical jargon unless necessary.
Example Answers
Linear regression is used to predict a continuous outcome, like predicting a person's weight based on height. Logistic regression, on the other hand, is used for predicting a binary outcome, such as whether an email is spam or not. For example, we could use linear regression to estimate a house price, while logistic regression could help us determine if a customer will buy a product based on clicks.
What are some key principles you follow when creating data visualizations for statistical data?
How to Answer
- 1
Understand the audience and what insights they need from the data
- 2
Choose the right type of chart to represent the data effectively
- 3
Use clear and descriptive labels for axes and legends
- 4
Maintain a clean and uncluttered design with appropriate color usage
- 5
Ensure that the data is accurate and the visualization supports your findings.
Example Answers
I focus on understanding my audience first, ensuring the visualization answers their specific questions. For example, when showing trends over time, I would choose a line chart that clearly illustrates these changes, and always label my axes and data points clearly so they are easy to interpret.
Explain the difference between stratified sampling and cluster sampling. In what situations would you use each method?
How to Answer
- 1
Define each sampling method clearly
- 2
Highlight the key differences between the methods
- 3
Provide practical examples of when to use each
- 4
Keep the explanation concise and focused
- 5
Use simple language to ensure clarity
Example Answers
Stratified sampling involves dividing the population into subgroups (strata) and taking samples from each. This method is ideal when the population has distinct subgroups and you want to ensure representation from each group. Cluster sampling, on the other hand, involves dividing the population into clusters and randomly selecting whole clusters. This is useful when it's difficult to reach every individual, and you need to save time and resources.
Can you explain the characteristics of a normal distribution and why it's important in statistics?
How to Answer
- 1
Define normal distribution and its shape (bell curve).
- 2
Mention key characteristics like mean, median, mode are equal.
- 3
Discuss standard deviation and its role in spread.
- 4
Explain the empirical rule (68-95-99.7) and its significance.
- 5
Highlight its importance in inferential statistics and hypothesis testing.
Example Answers
A normal distribution is a symmetric bell-shaped curve where the mean, median, and mode are all equal. The spread of the data is indicated by the standard deviation, and due to its properties, it allows for the use of the empirical rule, which facilitates predictions about data within one, two, and three standard deviations from the mean.
What techniques do you use for time series analysis and forecasting?
How to Answer
- 1
Start by mentioning key techniques like ARIMA and exponential smoothing.
- 2
Discuss the importance of seasonal decomposition in understanding trends.
- 3
Highlight how machine learning methods can be applied to improve forecasting accuracy.
- 4
Mention the role of cross-validation in validating your models.
- 5
Conclude with any software or tools you commonly use for these analyses.
Example Answers
I typically use ARIMA models for their effectiveness in capturing autocorrelations in time series data. Additionally, I apply seasonal decomposition to identify trends and seasonality before fitting models.
How do you integrate statistical models with machine learning algorithms in your analyses?
How to Answer
- 1
Identify the objectives of your analysis and choose appropriate statistical models
- 2
Use statistical methods to validate and interpret results from machine learning models
- 3
Apply machine learning algorithms to enhance predictive power of statistical models
- 4
Ensure that the assumptions of statistical models are satisfied in the data used for machine learning
- 5
Regularly assess model performance using statistical metrics and adjust accordingly
Example Answers
I start by defining the goals of my analysis and selecting the right statistical models that fit the data. For example, I might use regression models for forecasting and then employ machine learning algorithms to capture more complex patterns in the data. After training, I validate the machine learning model's performance against my statistical baseline.
What is ANOVA and how is it used in statistical analysis?
How to Answer
- 1
Define ANOVA clearly and mention what it stands for.
- 2
Explain the purpose of ANOVA in comparing multiple groups.
- 3
Mention types of ANOVA like one-way and two-way.
- 4
Provide an example of a situation where ANOVA would be applicable.
- 5
Summarize the output of ANOVA and how to interpret it.
Example Answers
ANOVA stands for Analysis of Variance. It is used to compare means across three or more groups to see if at least one mean is different from the others. For example, we might use it to compare test scores from students in different teaching methods. A one-way ANOVA tests for differences among the means of various groups based on one factor.
What is a Monte Carlo simulation and in what types of problems is it most useful?
How to Answer
- 1
Define Monte Carlo simulation clearly and simply.
- 2
Mention the role of randomness in the simulation process.
- 3
Explain its usefulness in estimating probabilities and outcomes.
- 4
Provide examples of problems where it is applicable, like finance or risk assessment.
- 5
Conclude with a statement on its importance in decision-making under uncertainty.
Example Answers
A Monte Carlo simulation is a computational technique that uses random sampling to estimate complex mathematical functions. It's particularly useful for problems involving uncertainty, such as financial forecasting or risk assessment, because it helps model and understand potential outcomes by simulating a wide range of possible scenarios.
Don't Just Read Mathematical Statistician Questions - Practice Answering Them!
Reading helps, but actual practice is what gets you hired. Our AI feedback system helps you improve your Mathematical Statistician interview answers in real-time.
Personalized feedback
Unlimited practice
Used by hundreds of successful candidates
When would you use a non-parametric test, and can you provide an example of such a test?
How to Answer
- 1
Identify situations where data does not meet parametric test assumptions.
- 2
Highlight the benefits of non-parametric tests, such as fewer assumptions.
- 3
Use specific examples to illustrate your point clearly.
- 4
Mention well-known non-parametric tests like the Mann-Whitney U test or Kruskal-Wallis test.
- 5
Explain the context in which these tests are preferred over parametric tests.
Example Answers
You would use a non-parametric test when the data does not follow a normal distribution or when you have ordinal data. An example of such a test is the Mann-Whitney U test, which compares the medians of two independent groups.
How do you approach statistical consulting for a project involving a domain you're not familiar with?
How to Answer
- 1
Start by conducting preliminary research on the industry and domain to gather basic knowledge
- 2
Identify key stakeholders and communicate with them to understand their goals and needs
- 3
Leverage your statistical knowledge to propose methodologies that apply broadly across domains
- 4
Collaborate with subject matter experts to gain insights and validate your statistical approaches
- 5
Stay adaptable and open to learning as the project progresses to refine your understanding
Example Answers
I begin by researching the domain to familiarize myself with its terminology and challenges. Then, I consult with stakeholders to grasp their main objectives and data sources. This, coupled with my statistical expertise, allows me to shape a robust methodology that meets their needs.
Explain the principles behind Bayesian statistics and how it differs from traditional frequentist approaches.
How to Answer
- 1
Define Bayesian statistics and its focus on prior knowledge and evidence.
- 2
Contrast it with frequentist statistics which relies solely on the data at hand without prior beliefs.
- 3
Mention the importance of the Bayes' theorem in updating probabilities with new evidence.
- 4
Discuss real-world applications where Bayesian methods are advantageous over frequentist methods.
- 5
Use clear examples to illustrate the differences in approach between the two methodologies.
Example Answers
Bayesian statistics allows us to incorporate prior beliefs into our analysis through Bayes' theorem. Unlike frequentist methods, which only use the data collected, Bayesian methods update these beliefs as new data becomes available, making them more flexible and informative in uncertain situations.
Situational Interview Questions
You find out that the dataset you've been using for analysis has some missing values. How would you handle this situation to ensure the integrity of your analysis?
How to Answer
- 1
Identify the pattern of the missing data to understand its nature
- 2
Consider using imputation methods if data is missing at random
- 3
If data is missing not at random, discuss the potential bias and implications
- 4
Ensure to conduct sensitivity analysis to see how missing values affect results
- 5
Clearly document the strategy used to address missing values for transparency
Example Answers
First, I would analyze the pattern of missing values to determine if they are random. If they're missing at random, I might use mean or median imputation. If not, I would document the potential biases this introduces in my analysis.
If a client is skeptical of the results from your statistical model, how would you present the data to reassure them of its validity?
How to Answer
- 1
Start with a clear summary of the model's purpose and methodology.
- 2
Use visualizations to demonstrate key results and confidence intervals.
- 3
Explain the data sources and the assumptions made in the model.
- 4
Provide examples of how the model's predictions have been validated with real outcomes.
- 5
Encourage questions and be open to discussing their concerns in detail.
Example Answers
I would begin by explaining the model's purpose and how it uses data. Then, I would show visualizations of the results, including confidence intervals, to demonstrate the reliability. I would also discuss the data sources used and mention validation with past outcomes.
Don't Just Read Mathematical Statistician Questions - Practice Answering Them!
Reading helps, but actual practice is what gets you hired. Our AI feedback system helps you improve your Mathematical Statistician interview answers in real-time.
Personalized feedback
Unlimited practice
Used by hundreds of successful candidates
You're working on a project with engineers and economists, each with different priorities. How do you balance and integrate their input into your statistical work?
How to Answer
- 1
Start by establishing clear communication with both teams.
- 2
Identify the key priorities and concerns from engineers and economists.
- 3
Facilitate joint meetings to encourage collaboration and understanding.
- 4
Utilize statistical methods that can satisfy both teams' needs.
- 5
Provide regular updates and be open to feedback throughout the project.
Example Answers
I begin by scheduling regular meetings with both the engineers and economists to clarify their priorities. By facilitating discussions, I can ensure everyone's input is acknowledged and find common ground for our statistical analysis.
Imagine you run a statistical test expecting a significant result, but you find no significance. How would you handle this situation?
How to Answer
- 1
Acknowledge the result and remain calm
- 2
Review your methodology for any potential errors
- 3
Consider the power of your test and sample size
- 4
Reflect on the theoretical implications of the non-significant result
- 5
Communicate the findings clearly, framing them in context
Example Answers
I would start by accepting the result and analyzing it. I would check my methodology for potential errors. Then I’d evaluate the power of my test and consider if my sample size was adequate. Finally, I would discuss the implications of the result honestly.
You are given a tight deadline for a complex statistical analysis project. How would you prioritize your tasks and manage your time to ensure the project is completed successfully?
How to Answer
- 1
Break down the project into smaller, manageable tasks.
- 2
Identify the key tasks that have the highest impact on the project's outcome.
- 3
Set realistic deadlines for each task to keep yourself on track.
- 4
Communicate with stakeholders to manage expectations and any potential roadblocks.
- 5
Regularly review your progress and adjust your plan as necessary.
Example Answers
I would start by breaking the project into smaller tasks, prioritizing those that are critical to the analysis. Then, I'd allocate time slots for each task while leaving some buffer for unforeseen challenges. Regular updates to my supervisor would help in managing expectations.
What steps would you take if you were asked to manipulate statistical findings for a study to support management's predetermined outcomes?
How to Answer
- 1
Prioritize integrity and ethical standards in your response.
- 2
Discuss the importance of accurate representation of data.
- 3
Mention the potential consequences of manipulating findings.
- 4
Highlight adherence to ethical guidelines in statistics.
- 5
Emphasize the role of transparency in statistical reporting.
Example Answers
I would refuse to manipulate the data, as integrity is crucial in statistics. Misrepresenting findings not only harms the credibility of the study but can also lead to negative consequences for the organization.
A dataset provided for analysis is messy and unstructured. How would you begin the process of cleaning and structuring this data for analysis?
How to Answer
- 1
Start by understanding the dataset's context and the specific issues it presents.
- 2
Identify and handle missing values appropriately, using imputation or deletion based on the nature of the data.
- 3
Standardize formats for categorical and date variables to ensure consistency.
- 4
Remove duplicates and irrelevant features that do not contribute to the analysis.
- 5
Consider transforming variables or creating new ones to make patterns more apparent during analysis.
Example Answers
First, I would assess the dataset to understand its structure and identify issues like missing values or inconsistencies. I would then apply techniques for handling missing values such as imputing or removing them as needed.
A stakeholder requests a custom statistical report with specific metrics that your current system doesn't support. How would you handle this request?
How to Answer
- 1
Clarify the specific metrics the stakeholder needs.
- 2
Assess the feasibility of creating the report with current tools.
- 3
Discuss alternative approaches or workarounds if necessary.
- 4
Set realistic timelines for report delivery.
- 5
Communicate regularly with the stakeholder throughout the process.
Example Answers
First, I would meet with the stakeholder to clarify exactly what metrics they need and understand their objectives. Then, I would evaluate our current reporting tools to see what can be done within those constraints. If those tools are inadequate, I would explore alternative methods, such as using a different software or potentially collaborating with IT for custom solutions. Lastly, I would communicate a realistic timeline for delivery and keep them updated on our progress.
How would you assess the potential benefits and challenges of adopting a new statistical technology or platform into your team’s workflow?
How to Answer
- 1
Identify specific benefits such as increased efficiency or improved accuracy.
- 2
Consider the learning curve and training requirements for the team.
- 3
Evaluate integration with existing tools and systems to avoid disruptions.
- 4
Assess any costs associated with the new technology versus the expected gains.
- 5
Gather feedback from team members to address concerns and insights.
Example Answers
I would start by analyzing how the new platform could streamline our data analysis process, which might increase our overall productivity. I'd also assess the cost of the platform versus potential improvements in accuracy from using advanced statistical methods. It's important to ensure that our team is comfortable with the new technology, so I would discuss training needs and integration with our current setups.
Mathematical Statistician Position Details
Recommended Job Boards
MathJobs
www.mathjobs.org/jobsCareerBuilder
www.careerbuilder.com/jobs/mathematical-statisticianThese job boards are ranked by relevance for this position.
Related Positions
Ace Your Next Interview!
Practice with AI feedback & get hired faster
Personalized feedback
Used by hundreds of successful candidates
Ace Your Next Interview!
Practice with AI feedback & get hired faster
Personalized feedback
Used by hundreds of successful candidates