Top 29 Applied Scientist Interview Questions and Answers [Updated 2025]
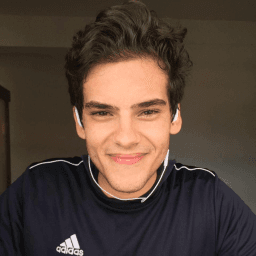
Andre Mendes
•
March 30, 2025
Preparing for an Applied Scientist interview can be daunting, but mastering the most common questions can set you on the path to success. In this post, we provide a curated list of essential interview questions for the Applied Scientist role, complete with example answers and practical tips. Dive in to enhance your responses and boost your confidence, ensuring you're ready to impress your interviewers.
Download Applied Scientist Interview Questions in PDF
To make your preparation even more convenient, we've compiled all these top Applied Scientistinterview questions and answers into a handy PDF.
Click the button below to download the PDF and have easy access to these essential questions anytime, anywhere:
List of Applied Scientist Interview Questions
Behavioral Interview Questions
Can you describe a time when you had to tackle a complex problem using your analytical skills?
How to Answer
- 1
Identify a specific problem where you used analysis.
- 2
Explain the data or methods you used to analyze the issue.
- 3
Describe the outcome and how your analysis contributed to it.
- 4
Focus on your thought process and decision-making.
- 5
Keep it concise and clear to maintain engagement.
Example Answers
In my previous role, we faced a drop in customer satisfaction. I collected feedback data and performed a regression analysis to find key pain points. After identifying the main issues, I proposed targeted changes, which improved our ratings by 25%.
Tell me about a successful collaboration you had with a cross-functional team. What was your role?
How to Answer
- 1
Choose a specific project that highlights your collaboration skills
- 2
Clearly define your role and responsibilities within the team
- 3
Explain the impact of the collaboration on the project's success
- 4
Mention how you overcame any challenges during the collaboration
- 5
Use metrics or outcomes to illustrate the success of the collaboration
Example Answers
In a project to develop a new recommendation system, I worked with engineers and product managers. I led the data analysis efforts, aligning our findings with business goals. Our collaboration increased user engagement by 30%, exceeding expectations.
Don't Just Read Applied Scientist Questions - Practice Answering Them!
Reading helps, but actual practice is what gets you hired. Our AI feedback system helps you improve your Applied Scientist interview answers in real-time.
Personalized feedback
Unlimited practice
Used by hundreds of successful candidates
Describe a situation where you had to lead a project. How did you motivate your team?
How to Answer
- 1
Start with a clear project context and your role as the leader
- 2
Highlight specific challenges the team faced
- 3
Describe the motivational strategies you used, such as setting goals or celebrating small wins
- 4
Mention how you fostered open communication and collaboration
- 5
Conclude with the project outcome and any lessons learned
Example Answers
In my last project, I led a team of data scientists to develop a predictive model for client retention. We faced tight deadlines and initial model inaccuracies. I motivated the team by setting weekly milestones and highlighting our progress. We also held daily stand-up meetings for open communication. Ultimately, we delivered a model that improved retention rates by 15%.
Give an example of a time when you had to adapt to significant changes at work. How did you manage?
How to Answer
- 1
Identify a specific change you faced and why it was significant
- 2
Explain your initial reaction and feelings about the change
- 3
Describe the steps you took to adapt and what strategies you employed
- 4
Highlight any support you sought or collaboration with others
- 5
Conclude with the outcome and what you learned from the experience
Example Answers
When our team switched to a remote work model during the pandemic, I initially felt overwhelmed. I took the initiative to set up daily check-ins with my colleagues to maintain communication. I also adapted my workflow by using new project management tools which improved our collaboration. As a result, our team met all deadlines and maintained productivity.
Can you provide an example of how you explained a complex scientific concept to a non-technical audience?
How to Answer
- 1
Choose a specific concept you explained.
- 2
Identify your audience and their level of understanding.
- 3
Use relatable analogies or examples to simplify the concept.
- 4
Focus on the key message or takeaway.
- 5
Encourage questions to ensure understanding.
Example Answers
I explained the concept of machine learning to a group of marketing professionals by comparing it to a recipe. I said just like how a recipe requires specific ingredients and steps to make a dish, machine learning involves data and algorithms that learn patterns to make predictions. They related well and asked insightful questions afterward.
Describe a time when you faced a conflict in a project. How did you resolve it?
How to Answer
- 1
Identify the specific conflict clearly and concisely.
- 2
Explain your role in the situation and how it impacted the project.
- 3
Describe the steps you took to resolve the conflict.
- 4
Highlight any communication strategies or tools you used.
- 5
Share the final outcome and what you learned from the experience.
Example Answers
In a project to develop a new machine learning model, my team disagreed on the choice of algorithms. I facilitated a meeting where each member presented their reasoning. We decided to run tests on both options, which led us to choose the most effective algorithm. This experience taught me the importance of collaborative decision-making.
What steps have you taken to further your skills and knowledge within your field?
How to Answer
- 1
Describe specific online courses or certifications you've completed in relevant technologies.
- 2
Mention any projects or research you've done that applied new methodologies.
- 3
Talk about attending workshops or conferences to stay updated on industry trends.
- 4
Share experiences of collaborating with peers or joining study groups.
- 5
Explain how you engage with professional communities or contribute to forums.
Example Answers
I completed a deep learning specialization on Coursera, which enhanced my understanding of neural networks. I've also worked on a personal project applying these techniques to image classification.
Have you ever mentored someone? What was your approach and what did you learn from the experience?
How to Answer
- 1
Choose a specific mentoring experience and describe it briefly
- 2
Highlight the methods you used, like regular check-ins or setting goals
- 3
Share what the mentee achieved to demonstrate impact
- 4
Explain personal lessons learned, such as communication or adaptability
- 5
Conclude with how it shaped your approach to collaboration in future projects
Example Answers
I mentored a junior data scientist on a project about predictive modeling. I scheduled weekly check-ins to discuss challenges and set milestones. The mentee successfully built a model that increased accuracy by 15%. I learned the importance of tailoring my guidance to individual learning styles.
Describe a project you were proud of. How did you measure its success?
How to Answer
- 1
Choose a project that showcases your skills and achievements.
- 2
Explain your specific role and contributions clearly.
- 3
Include measurable outcomes or metrics to define success.
- 4
Highlight any challenges faced and how you overcame them.
- 5
Connect the project's impact to the organization's goals.
Example Answers
I led a machine learning project that improved customer retention by 20%. I used A/B testing to measure the success of the new recommendation system I developed. This directly increased sales and was praised by management.
Technical Interview Questions
What are the differences between supervised and unsupervised learning? Can you provide examples of each?
How to Answer
- 1
Define supervised learning with an emphasis on labeled data.
- 2
Define unsupervised learning and focus on unlabeled data.
- 3
Explain the goal of each learning type clearly.
- 4
Provide a specific example for each type to illustrate your points.
- 5
Keep the explanation concise and avoid technical jargon.
Example Answers
Supervised learning uses labeled data to train models, aiming to predict outcomes. For example, predicting house prices based on features like size and location. In contrast, unsupervised learning deals with unlabeled data, seeking to find patterns. An example is clustering customers based on purchasing behavior without predefined categories.
Which tools and methodologies do you use for data analysis? Why do you prefer them?
How to Answer
- 1
Identify specific tools you use, like Python, R, or SQL.
- 2
Mention methodologies such as regression analysis, machine learning, or A/B testing.
- 3
Explain your preference based on usability, community support, or performance.
- 4
Relate your answer to the job requirements and how these tools help you solve relevant problems.
- 5
Use examples from past experiences to illustrate your points.
Example Answers
I primarily use Python with libraries like Pandas and NumPy for data manipulation. I prefer Python due to its strong community support and versatility in tasks ranging from data cleaning to building machine learning models, which aligns perfectly with the responsibilities of this role.
Don't Just Read Applied Scientist Questions - Practice Answering Them!
Reading helps, but actual practice is what gets you hired. Our AI feedback system helps you improve your Applied Scientist interview answers in real-time.
Personalized feedback
Unlimited practice
Used by hundreds of successful candidates
Can you write a simple code snippet in Python to perform linear regression?
How to Answer
- 1
Import necessary libraries like pandas and scikit-learn
- 2
Prepare your dataset by defining independent and dependent variables
- 3
Create a linear regression model instance
- 4
Fit the model with your data
- 5
Output the model's coefficients or predictions
Example Answers
import pandas as pd from sklearn.linear_model import LinearRegression data = {'X': [1, 2, 3, 4, 5], 'Y': [2, 3, 5, 7, 11]} df = pd.DataFrame(data) model = LinearRegression() model.fit(df[['X']], df['Y']) print(model.coef_, model.intercept_)
How do you determine if a dataset is normally distributed?
How to Answer
- 1
Visualize the data using a histogram or QQ plot to assess the shape.
- 2
Calculate descriptive statistics like mean, median, and standard deviation.
- 3
Use statistical tests such as the Shapiro-Wilk test or Kolmogorov-Smirnov test.
- 4
Check skewness and kurtosis values for normality indicators.
- 5
Consider sample size; larger datasets may show normality more reliably.
Example Answers
To determine if a dataset is normally distributed, I usually start by visualizing the data with a histogram and a QQ plot. This immediately lets me see if the data follows a bell-shaped curve. Next, I calculate the mean and median to check their proximity. If I need further analysis, I apply the Shapiro-Wilk test for statistical confirmation.
What metrics do you use to evaluate the performance of a machine learning model?
How to Answer
- 1
Identify the type of problem: classification, regression, or clustering.
- 2
Mention common metrics used for each problem type.
- 3
Include examples of why specific metrics are relevant.
- 4
Discuss the importance of baseline models for comparison.
- 5
Consider trade-offs between precision and recall if applicable.
Example Answers
For classification tasks, I often use accuracy, precision, recall, and F1-score. For example, in a medical diagnosis model, precision is crucial to minimize false positives.
Can you explain what a convolutional neural network is and when it might be used?
How to Answer
- 1
Define convolutional neural networks (CNNs) clearly and simply.
- 2
Mention the layers involved, such as convolutional and pooling layers.
- 3
Explain their primary use in image processing and computer vision.
- 4
Provide examples of tasks where CNNs are effective, like image classification or object detection.
- 5
Conclude with a statement about their importance in the field of deep learning.
Example Answers
A convolutional neural network is a type of deep learning model designed to process data with a grid-like topology, such as images. It consists of layers that perform convolution operations, followed by pooling layers that down-sample the data. CNNs are mainly used for tasks like image recognition and object detection because they automatically learn spatial hierarchies of features.
How do you approach the challenges of working with big data?
How to Answer
- 1
Identify the specific big data challenges you have faced, such as storage, processing, or analysis.
- 2
Discuss tools or techniques, like Hadoop or Spark, you use to manage large datasets effectively.
- 3
Emphasize the importance of data quality and how you ensure it during processing.
- 4
Mention collaboration with data engineers and stakeholders to align on data strategy.
- 5
Include examples of projects where you successfully derived insights from big data.
Example Answers
In my previous role, I tackled big data challenges by using Spark for processing large datasets. I ensured data quality by implementing validation checks and collaborated closely with data engineers to optimize our data architecture.
What strategies do you employ for feature selection and engineering?
How to Answer
- 1
Start with domain knowledge to identify potentially relevant features
- 2
Use statistical methods like correlation matrices to evaluate feature importance
- 3
Apply dimensionality reduction techniques such as PCA to simplify complex datasets
- 4
Experiment with feature transformation techniques like normalization or encoding
- 5
Iteratively refine feature sets based on model performance metrics
Example Answers
I begin by leveraging domain expertise to highlight key features, then use correlation matrices to identify their importance. For high-dimensional data, I apply PCA to reduce complexity, followed by testing different feature transformations to see how they affect model accuracy.
How do you choose which algorithm to use for a particular problem?
How to Answer
- 1
Identify the problem type: classification, regression, clustering, etc.
- 2
Evaluate dataset size and quality: consider whether the data is structured or unstructured.
- 3
Assess the interpretability requirements: determine if model transparency is crucial.
- 4
Consider computational resources: analyze time constraints and available hardware.
- 5
Test and iterate: implement a few algorithms to compare performance and refine choices.
Example Answers
For classification tasks, I first check the size and balance of the dataset. If it's large and imbalanced, I might choose random forests for robustness. If interpretability is significant, I'd consider logistic regression.
What software development practices do you follow when building models?
How to Answer
- 1
Adopt version control to track changes and collaborate with team members
- 2
Document your code and model decisions to enhance understandability
- 3
Implement unit tests to ensure model reliability and performance
- 4
Use a modular coding approach to facilitate reusability and maintenance
- 5
Regularly review and refactor code as needed to improve quality
Example Answers
I follow version control practices using Git to manage code and collaborate effectively. I document my model choices and code, which makes it easier for others to understand and contribute.
Don't Just Read Applied Scientist Questions - Practice Answering Them!
Reading helps, but actual practice is what gets you hired. Our AI feedback system helps you improve your Applied Scientist interview answers in real-time.
Personalized feedback
Unlimited practice
Used by hundreds of successful candidates
Situational Interview Questions
If you find that the data you've been provided is inconsistent and unreliable, what steps would you take?
How to Answer
- 1
Identify specific inconsistencies in the data.
- 2
Consult with stakeholders to understand the data sources.
- 3
Determine the impact of the inconsistencies on your analysis.
- 4
Consider alternative data sources or methods for imputation.
- 5
Document your findings and proposed solutions.
Example Answers
First, I would pinpoint the exact inconsistencies in the data, such as missing values or outliers. Then, I would reach out to stakeholders to clarify the data sources. This helps assess the impact of the issues on my analysis. If necessary, I would explore alternative datasets or methods to fill in gaps, and I would document all my findings and the steps taken.
Imagine you are leading a project with a tight deadline. How would you prioritize tasks?
How to Answer
- 1
Identify key deliverables that directly impact project goals
- 2
Assess dependencies to understand task order and priority
- 3
Evaluate the time needed for each task and allocate resources effectively
- 4
Communicate priorities with the team to align efforts
- 5
Regularly review progress and adjust priorities as needed
Example Answers
I would first identify the key deliverables and break the project into main tasks. Then, I'd assess which tasks have dependencies and need to be completed first. I'd allocate resources based on the estimated time each task would take, keeping the team informed throughout the process. Regular check-ins would help adapt our priorities if any issues arise.
Don't Just Read Applied Scientist Questions - Practice Answering Them!
Reading helps, but actual practice is what gets you hired. Our AI feedback system helps you improve your Applied Scientist interview answers in real-time.
Personalized feedback
Unlimited practice
Used by hundreds of successful candidates
How would you approach a situation where stakeholders have conflicting requirements for a project?
How to Answer
- 1
Identify all stakeholders and their specific requirements.
- 2
Facilitate a meeting to discuss and understand the rationale behind each requirement.
- 3
Prioritize the requirements based on overall project goals and stakeholder impact.
- 4
Propose alternative solutions or compromises that meet the majority of needs.
- 5
Document and communicate the agreed-upon requirements and the reasoning behind decisions.
Example Answers
I would start by mapping out all stakeholder requirements and then gather everyone for a meeting to discuss their reasoning. After understanding their perspectives, I would prioritize the requirements based on project goals and look for compromises that address most concerns.
What would you do if you discovered that an algorithm you developed had a bias?
How to Answer
- 1
Acknowledge the bias and its potential impact on users
- 2
Investigate the source of the bias through data analysis
- 3
Collaborate with stakeholders to discuss findings
- 4
Redesign the algorithm to mitigate bias and improve fairness
- 5
Implement ongoing monitoring to catch future biases early
Example Answers
I would first acknowledge the bias and understand how it affects the users. Next, I'd analyze the data to find its source. Then, I would meet with my team to present the findings and propose a redesign to minimize the bias, ensuring we also set up a monitoring process for the future.
You are tasked with improving an existing model that is not performing well. What approach would you take?
How to Answer
- 1
Analyze model performance metrics to identify weaknesses
- 2
Research data quality and feature relevance
- 3
Experiment with hyperparameter tuning
- 4
Consider alternative algorithms or ensemble methods
- 5
Implement cross-validation to ensure robustness
Example Answers
First, I would review the performance metrics to locate specific issues. Next, I would assess the data quality, checking for missing values or outliers. Based on my findings, I might try different features or hyperparameter tuning for optimization.
If a deployed model fails to deliver expected results, how would you go about diagnosing the issue?
How to Answer
- 1
Check data quality and distribution changes since model training
- 2
Review model performance metrics to identify deviations
- 3
Analyze feature importance to determine unexpected influences
- 4
Look for biases in the input data or model predictions
- 5
Consult logs or monitoring systems for runtime errors or anomalies
Example Answers
First, I would evaluate the incoming data for any discrepancies or changes in its distribution compared to the training set. Then, I'd analyze the performance metrics to spot where the model is underperforming.
You have limited resources for a project. How would you ensure successful delivery within those constraints?
How to Answer
- 1
Identify core project goals and prioritize tasks based on impact
- 2
Break down the project into smaller, manageable phases
- 3
Gather feedback regularly to make adjustments on-the-fly
- 4
Utilize existing tools and data to minimize resource use
- 5
Communicate transparently with stakeholders about limitations and progress
Example Answers
I would start by defining the project's critical objectives and prioritize the must-have features. Next, I would divide the work into smaller phases, allowing for regular feedback and adjustments to stay on track.
You receive critical feedback from a peer on your work. How would you address it?
How to Answer
- 1
Acknowledge the feedback without being defensive
- 2
Ask clarifying questions to understand the feedback better
- 3
Reflect on the feedback and consider its validity
- 4
Communicate appreciation for the feedback and any actionable next steps
- 5
Follow up with your peer after making changes to keep the dialogue open
Example Answers
I would thank my peer for their feedback, ask for specific examples to understand their perspective, and then reflect on how I can incorporate their suggestions into my work.
How would you handle multiple competing deadlines for different projects?
How to Answer
- 1
Prioritize tasks based on impact and urgency
- 2
Break projects into smaller, manageable tasks
- 3
Communicate clearly with stakeholders about timelines
- 4
Use tools to track progress and deadlines
- 5
Be flexible and adjust plans as necessary
Example Answers
I prioritize my tasks by assessing their urgency and impact, breaking each project down into smaller tasks, and setting clear milestones. I also keep stakeholders informed to manage expectations.
If asked to propose a new scientific approach to solve an existing problem, what steps would you take?
How to Answer
- 1
Identify the problem clearly and understand its context
- 2
Research existing solutions and their limitations
- 3
Brainstorm innovative approaches or technologies that could be applied
- 4
Outline a potential methodology for testing your idea
- 5
Consider the feasibility, scalability, and ethics of your proposed solution
Example Answers
I would start by defining the specific problem and reviewing current solutions. Then, I’d explore emerging technologies in the field that could create new pathways. I would draft a research plan that includes experimental methods and consider the resource implications before moving forward.
Don't Just Read Applied Scientist Questions - Practice Answering Them!
Reading helps, but actual practice is what gets you hired. Our AI feedback system helps you improve your Applied Scientist interview answers in real-time.
Personalized feedback
Unlimited practice
Used by hundreds of successful candidates
Applied Scientist Position Details
Salary Information
Recommended Job Boards
CareerBuilder
www.careerbuilder.com/jobs-applied-scientistZipRecruiter
www.ziprecruiter.com/Jobs/Applied-ScientistThese job boards are ranked by relevance for this position.
Related Positions
Ace Your Next Interview!
Practice with AI feedback & get hired faster
Personalized feedback
Used by hundreds of successful candidates
Ace Your Next Interview!
Practice with AI feedback & get hired faster
Personalized feedback
Used by hundreds of successful candidates